If you’re a commercial insurer, how do you evaluate your claims personnel? How do those evaluations impact your decisions on how to deploy individual adjusters?
Typically, adjusters are assigned by pre-determined categories such as the line of business, size of the account, or region. Within these categories, adjuster performance is measured by various metrics, including closed-claims ratio, average time to settlement, accuracy of loss development projections, and others.
This amounts to what might be called “input-based” claims management, where a claim is routed through a predetermined path based on limited information in the initial notice of loss. This approach has worked well enough to date, given the complexity of commercial claims management and the limitations–until now–on insurers’ ability to analyze loss development in real-time.
Artificial intelligence and machine learning are poised to turn claims management on its head, however, by allowing commercial insurers to implement “outcome-based” adjuster assignment –an approach that breaks down functional silos to identify the adjuster best prepared to reach an optimum settlement on an individual claim.
Claim Dynamics
To understand the value of outcome-based adjusting, it is important to remember that every claim has a unique dynamic of interacting variables that drive its loss development. These loss characteristics don’t neatly fall into our predetermined claims management categories.
For example, a worker’s comp claim involving a vehicle collision may have more in common with an auto claim than the typical worker’s comp claim. A seemingly small property loss can lead to a disproportionately large income loss if an otherwise skilled property adjuster is unaccustomed to the type of operation involved.
Similarly, the right person for managing a claim might not be where you would expect within a traditional claims operation. An adjuster specializing in artisan contractors may be at a loss to adjust an intellectual property claim over a design feature. In that case, how would the company know that the right adjuster for that type of claim is currently handling retail accounts?
An outcome-based assignment is based on a systematic and highly granular analysis of closed claims to determine how each adjuster has performed on each individual coverage subsegment. For comparable claims, each adjuster is measured for expense control, accuracy of case reserves, average claim cost, audit outcomes, customer satisfaction / NPS, and other metrics. In addition, AI and machine learning will identify cost factors and correlations that would typically go undetected.
From this, individual adjusters can be scored for their particular aptitudes, and the highest-scoring available adjuster for a specific type of claim can be assigned to such a claim. The most appropriate adjuster for a claim is not necessarily your best adjuster overall, or even the best for that line of business or class of risk, but is the best choice given the characteristics of the loss and the current demands on your claims team.
Optimizing Outcomes
The aspiration here is not new, but the capability is.
For years, commercial claims managers have sought to optimize adjuster assignments by personally deviating from organizational structure and assigning an adjuster to a claim, typically on the basis of intuitive insight gained from experience.
Today, adjusting competence can be determined on a very granular level and implemented consistently across the entire claims operation. Why make exceptions when you can make an optimal assignment the rule?
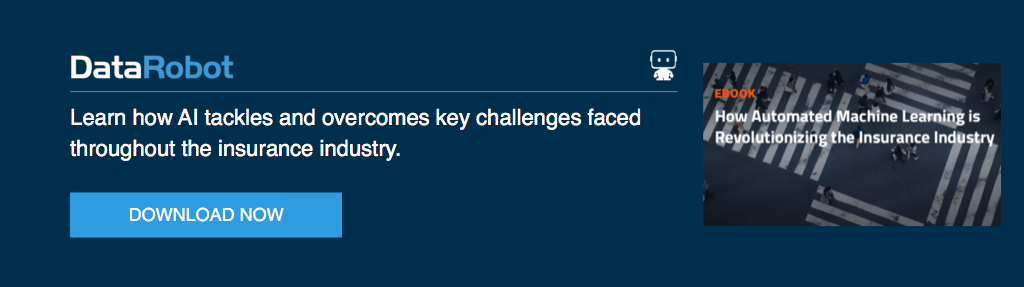
About the author
Neal Silbert
General Manager of Insurance, DataRobot
As an insurance industry executive and management consultant, Neal has served as an analytics thought leader and driver of innovation for the last 25 years. Recently, he was the VP of Predictive Analytics at Zurich North America, focusing on bringing the latest advances in predictive analytics to insurance product development.
Meet Neal Silbert