AI has made great impacts on organizations across all industries and around the world. As AI adoption increases, more use cases are beginning to emerge, especially within the healthcare industry. Looking over the past decade, we can see how many healthcare organizations have been transformed with regard to the way they use data. And, as healthcare groups continue to leverage AI throughout their teams and departments, they’ll need to focus on monitoring and management to prevent misuse and ensure success.
Most healthcare organizations are using data to make business and clinical decisions
The data revolution saw most payers, providers, and life science organizations become data-driven in the last decade. Typically, business intelligence teams deliver insights to decision-makers via automated data prep or ETL pipelines and dashboarding tools. All healthcare organizations have business intelligence functions, but now, many are also incorporating and leveraging AI throughout their processes.
Many organizations have matured their analytics and are now using AI to realize value in big ways. Some battle-tested AI use cases have become commonplace. These use cases include predicting: hospital admissions/readmissions and health condition risk, fraudulent claims, high-cost claimants and care plan or protocol adherence risk for life science, payers, and providers alike. More on this can be found here.
Mature healthcare organizations are starting to push AI use cases
These battle-tested use cases have proven valuable in improving outcomes, removing waste, and driving clinical and operational effectiveness. Now we’re starting to see these organizations stretch AI into different lines of business, attacking problems like predicting clinician churn, identifying case-related litigation risk, or using AI to optimize development operations teams to better deliver applications to patients and members.
So what does this mean?
With the broadening use of AI across Healthcare and Life Science enterprises, organizations need to plan for monitoring and management of their predictive models in order to prevent error and misuse. Additionally, careful planning is required to remove and monitor for bias in AI solutions. This is done through a combination of thoughtfully defining the use case, preparing the data, and using technologies with the right guardrails to help prevent building predictive models that are biased.
Mature healthcare organizations have the skills, the data, and the opportunity to use AI to drastically improve outcomes and provide value across the enterprise. It’s crucial to have the appropriate approach and technologies to ensure patient/member safety is not compromised by bias and inequality as AI is used more broadly.
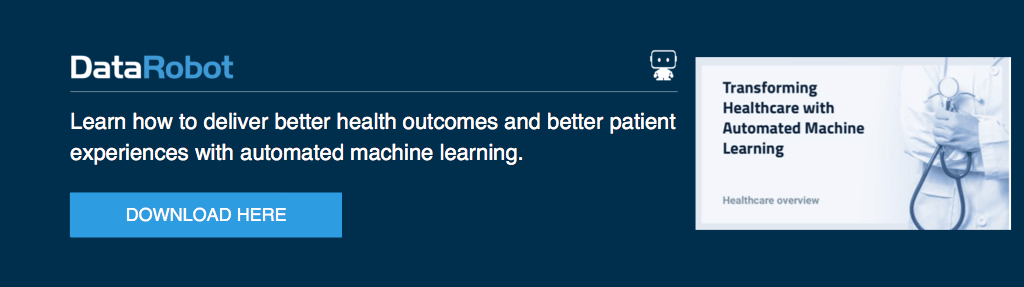
About the author
Matt Marzillo
Data Scientist
Matt Marzillo is a data scientist at DataRobot, based out of Chicago. He’s currently enabling customers with data science projects with a primary focus in healthcare, including payers, providers, and life science organizations. Prior to DataRobot Matt has worked with several healthcare companies both as an internal data science leader and as a consultant. Matt has an MS in Predictive Analytics from Northwestern University where he still holds an appointment as an Adjunct Instructor.
Meet Matt Marzillo