AI in Financial Markets Part 1: It Looks like Magic, But It’s Got to Be Real…
Join Peter Simon, DataRobot’s lead data scientist for financial markets, on part one of the podcast, AI in Financial Markets.
Guests

Peter Simon
Managing Director, Financial Markets Data Science
Related Resources
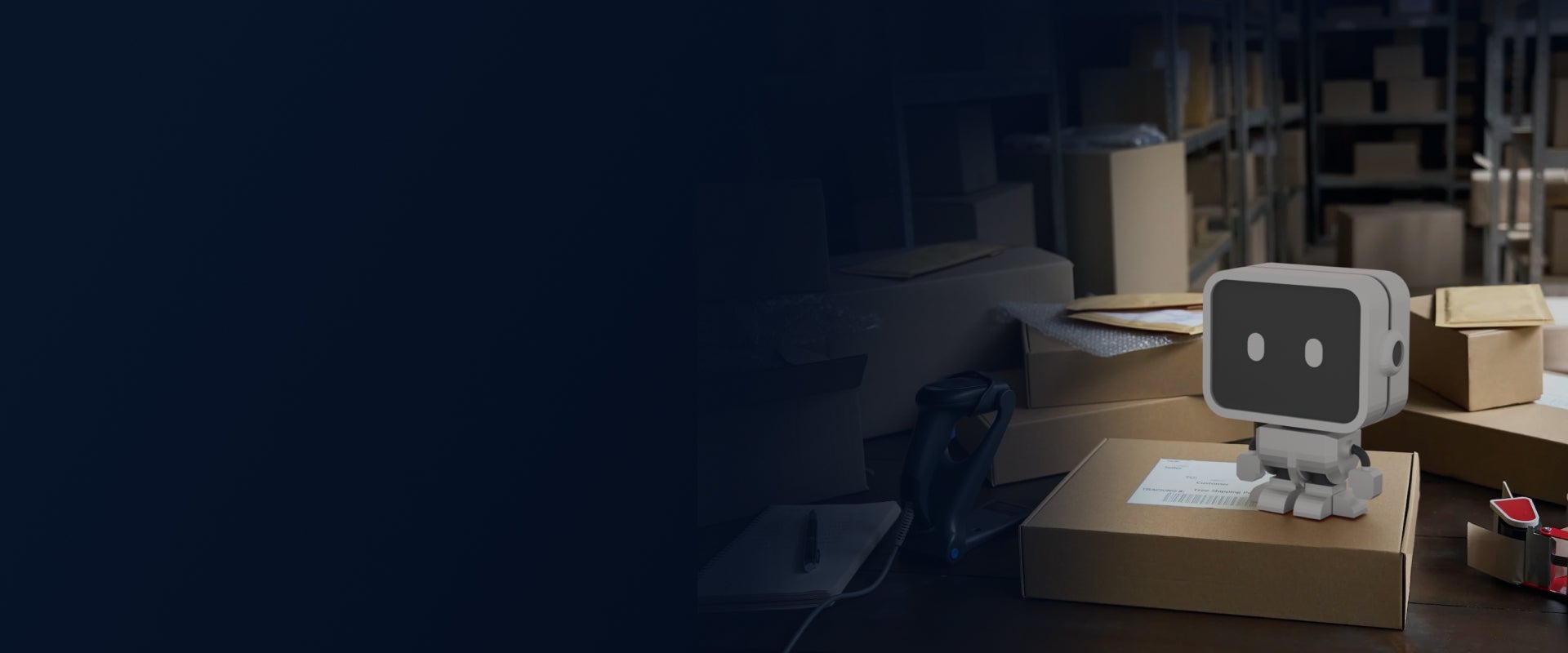