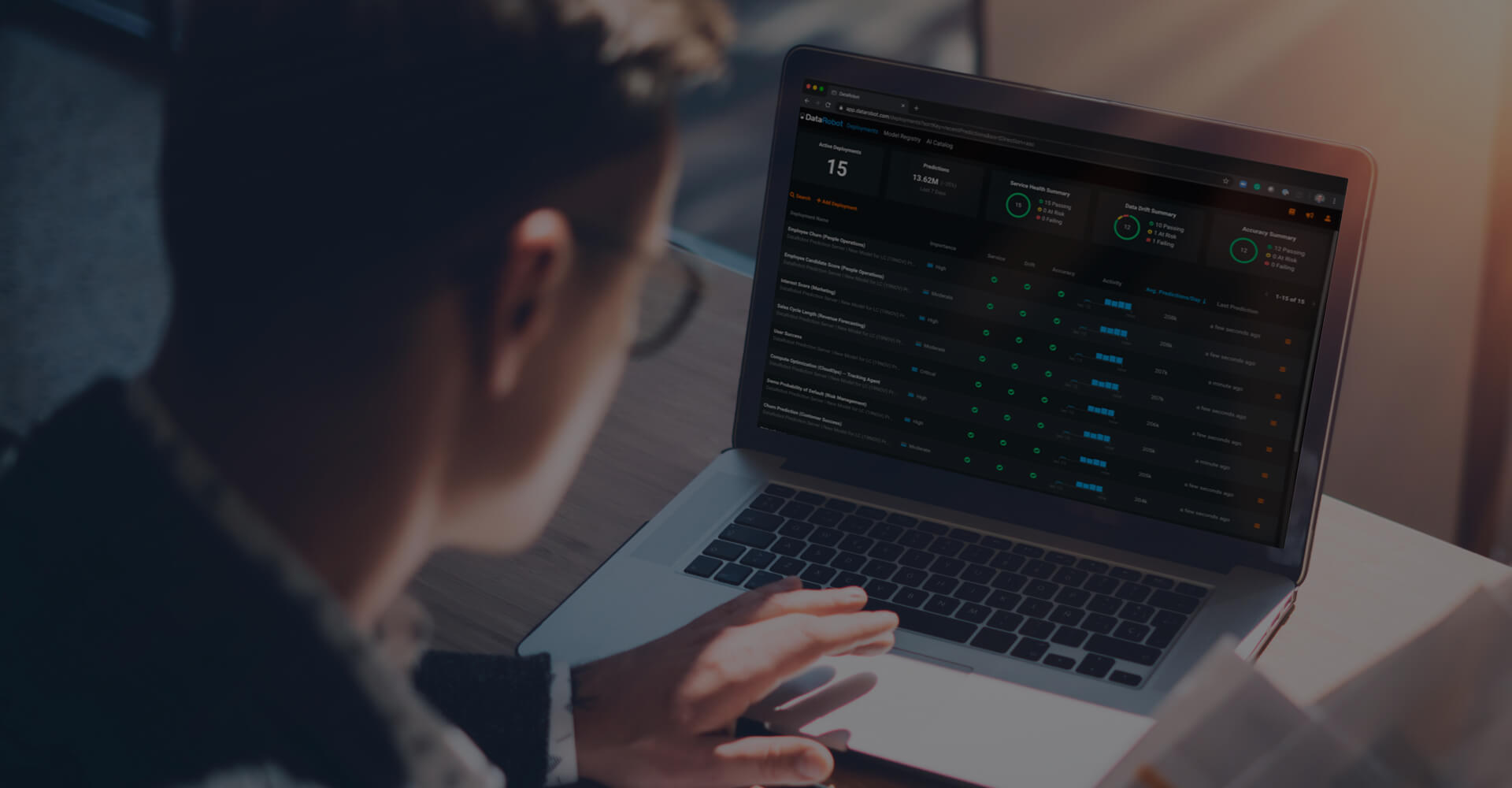
Who Needs MLOps?
The short answer is that everyone needs MLOps.
The market has matured to a point that machine learning models have to start showing and proving value. They must do this by monitoring for performance and accuracy in order to eliminate business risk. The path to achieving this is a scalable way to manage production machine learning.
Who is “Everyone”?
AI-based applications promise to deliver new levels of competitiveness, intelligence, and automation for businesses. Despite increasing investments in data science talent and technologies, few companies actually deploy their AI into production. Without the ability to automate the deployment of machine learning models in production, coupled by trusted ways to monitor, manage, and govern them, companies cannot generate measurable value from AI.
MLOps Can Help Those in the Following Roles:
Is MLOps Right for My Organization?
Organizations with multiple data science teams creating and attempting to deploy machine learning models need MLOps. Even if some models are running in production already, custom coding and manual processes cause roadblocks. Scaling is difficult, and DataRobot can help organizations build a governance strategy to get those models off the ground.
Request a DemoMLOps Serves:
- Organizations that are just getting started (<1-5 models in production). Data scientists who are frustrated with having to manually deploy, monitor and replace models by following IT processes that are not designed for machine learning.
- Organizations that are attempting to create processes for machine learning in production. Data science, data engineering, architects or IT personas tasked with improving processes for deploying, monitoring, and managing models in production.
- Mature organizations that have processes for updating models in production. Data science, IT, and MLOps leaders who are seeking a solution for increasing the speed and capacity of MLOps, often motivated by an executive who is frustrated that it takes way too long to deploy and replace a model.
MLOps Customers
Companies across every industry leverage DataRobot’s MLOps solution, such as:

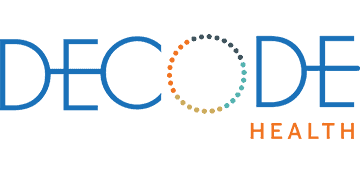
