Enterprise AI Suite
Agentic AI Apps
Develop, deliver, and govern custom agentic AI applications for your business needs
Tour the product


Don’t settle for generic AI.
Build custom agentic AI solutions that align with your business, protect your data, and seamlessly integrate with your everyday operations.
Agentic AI Apps
AI Consumers
Business Users
Executives
Customers
Supply Chain
Operations
Financial Planning & Accounting
Customer Experience
Sales
Credit & Risk
And much more
Agentic Flows
Data Analysis
Digital Assistants
Predictive Content Generation
Content Summarization
Supply chain & operations
Support an elastic supply chain with AI-powered forecasting and operational excellence.
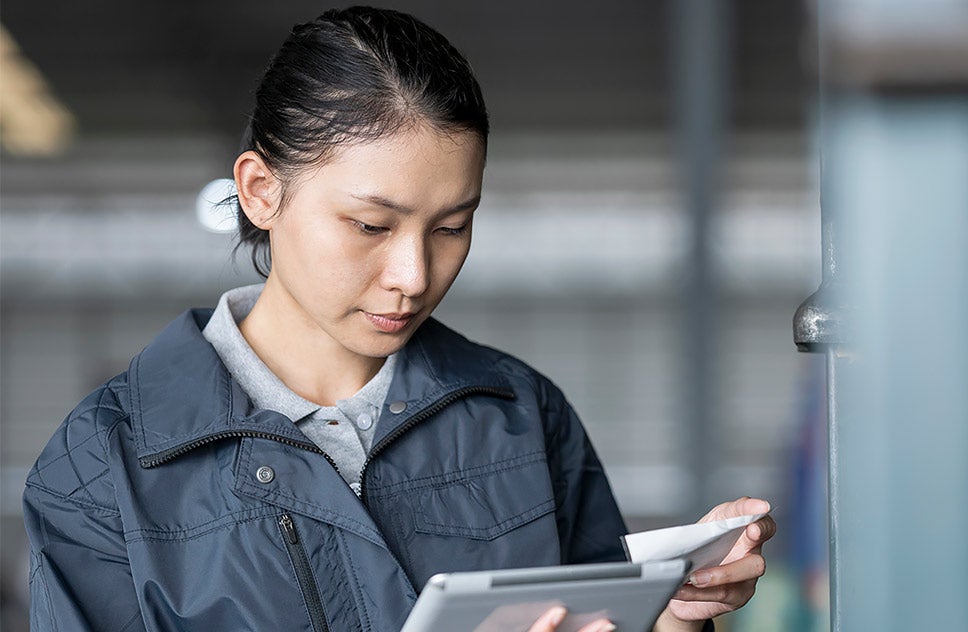
Generative AI
- Inventory optimization
- Materials processing efficiency
- Mechanical design optimization
- Sourcing optimization
- Product quality intelligence
- Warehouse resource management optimization
- On-demand operations dashboards
Predictive AI
- Demand forecasting
- New product forecasting
- Price optimization
- Shipment delay forecasting
- Stockout risk reduction
- Quality control
- Supplier counter offer propensity
Customer service
Deliver high-quality customer service with a portfolio of generative and predictive applications tailored to your business needs.
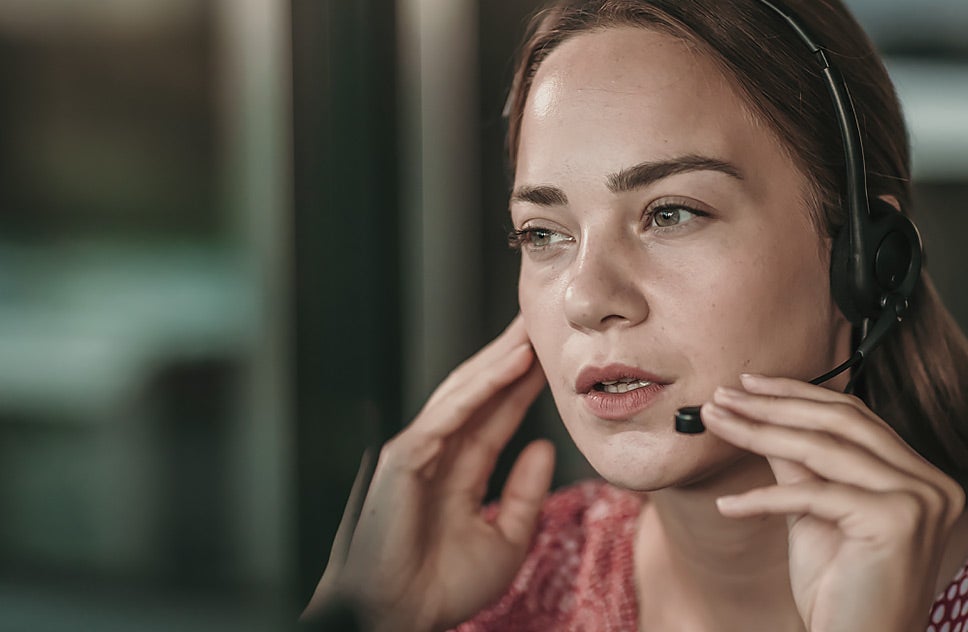
Generative AI
- Personalized communications
- Product recommendations
- Conversational chat interface
- Customer order substitution
Predictive AI
- Call center staffing
- Complaint management
- Customer lifetime value
- Customer satisfaction factor analysis
Financial planning & analysis
Adapt and embed AI across every facet of your financial planning and analytical processes with industry-leading models and components.
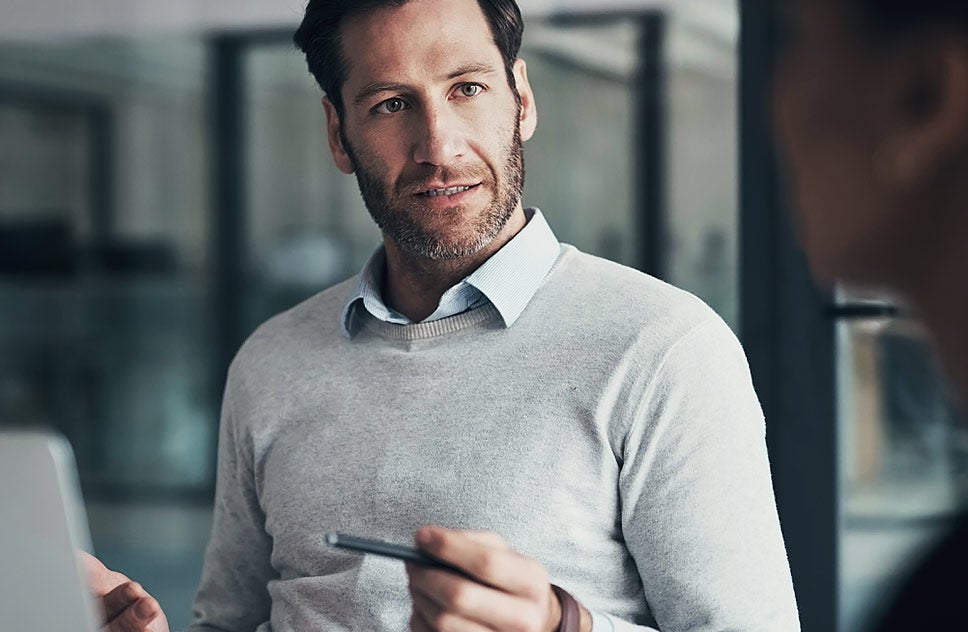
Generative AI
- Automated report generation
- Customized pricing models
- Dynamic financial modeling
- Vendor contract creation
- Scenario modeling
- Document analysis and processing
- Policy and procedure generation
Predictive AI
- Forecasting and budgeting
- Credit risk analysis
- Cash flow management
- Contract analysis
- Fraud detection
- What-if analysis
Sales & marketing
Optimize productivity, customer engagement, and revenue with AI-driven insights and experiences.
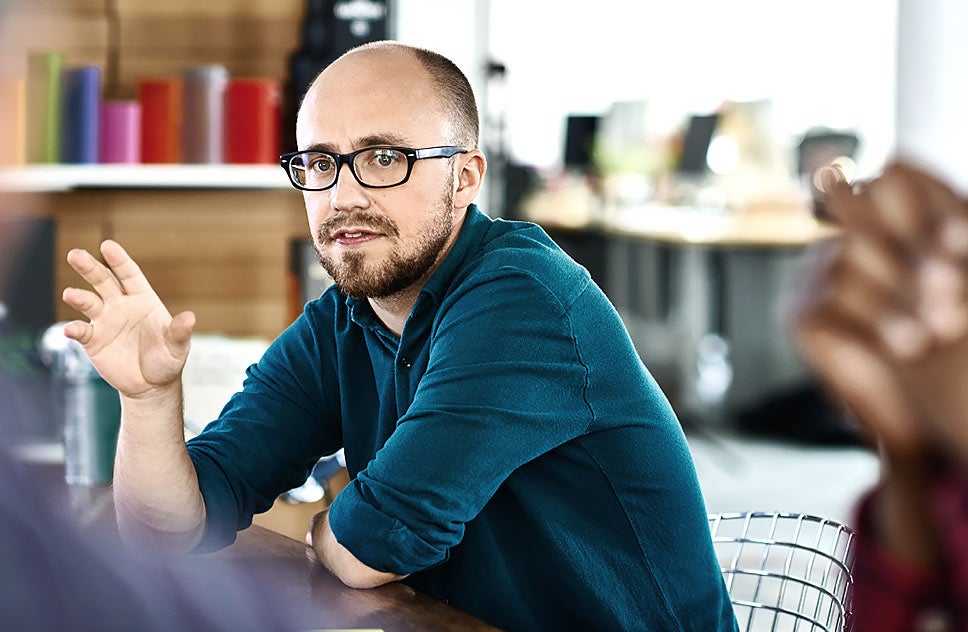
Generative AI
- Audience targeting
- Customized content generation
- Enhanced search and upselling
- Social media customer sentiment
- Customer behavior modeling
- Market segmentation
Predictive AI
- Demand forecasting
- Opportunity triage
- Churn prediction
- Lead scoring
- Revenue forecasting
- Marketing mix attribution
Credit risk
Automate and accelerate credit and risk analysis with AI models that drive timely action.
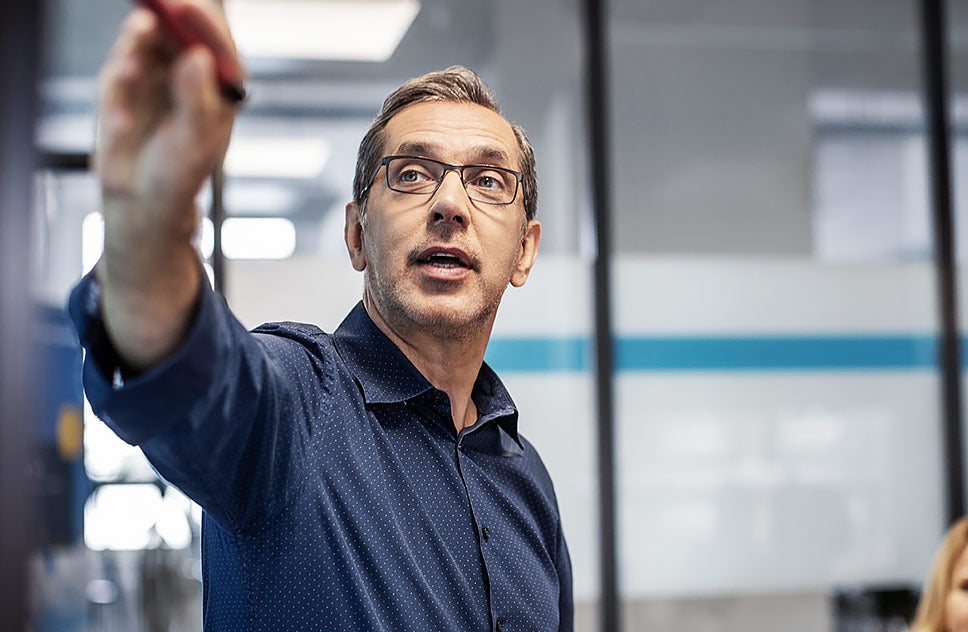
Generative AI
- Automated risk assessment reports
- AML alert scoring report
- Suspicious activity alerts
- Explanation generation
- Loans processing
- Debt collection and recovery assistant
- Credit decision document review
- Policy violation flagging
- Outreach communication generation
Predictive AI
- Credit risk and loss forecasting
- Credit risk modeling
- Prepayment risk
- Small business, commercial credit, and collateral valuation
- Loan default risk assessment
- Fraud detection
- Operational risk, process fails, false positives reduction
- Credit scoring and approval
- Price elasticity of demand
- Collections
Tour the product
Explore how DataRobot can help you create unique agentic AI applications your business will use every day.
Take a tour