Are you seeing tangible results from your investment in generative AI — or is it starting to feel like an expensive experiment?
For many AI leaders and engineers, it’s hard to prove business value, despite all their hard work. In a recent Omdia survey of over 5,000+ global enterprise IT practitioners, only 13% of have fully adopted GenAI technologies.
To quote Deloitte’s recent study, “The perennial question is: Why is this so hard?”
The answer is complex — but vendor lock-in, messy data infrastructure, and abandoned past investments are the top culprits. Deloitte found that at least one in three AI programs fail due to data challenges.
If your GenAI models are sitting unused (or underused), chances are it hasn’t been successfully integrated into your tech stack. This makes GenAI, for most brands, feel more like an exacerbation of the same challenges they saw with predictive AI than a solution.
Any given GenAI project contains a hefty mix of different versions, languages, models, and vector databases. And we all know that cobbling together 17 different AI tools and hoping for the best creates a hot mess infrastructure. It’s complex, slow, hard to use, and risky to govern.
Without a unified intelligence layer sitting on top of your core infrastructure, you’ll create bigger problems than the ones you’re trying to solve, even if you’re using a hyperscaler.
Here, I break down six tactics that will help you shift the focus from half-hearted prototyping to real-world value from GenAI.
6 Tactics That Replace Infrastructure Woes With GenAI Value
Incorporating generative AI into your existing systems isn’t just an infrastructure problem; it’s a business strategy problem—one that separates unrealized or broken prototypes from sustainable GenAI outcomes.
But if you’ve taken the time to invest in a unified intelligence layer, you can avoid unnecessary challenges and work with confidence. Most companies will bump into at least a handful of the obstacles detailed below. Here are my recommendations on how to turn these common pitfalls into growth accelerators:
1. Stay Flexible by Avoiding Vendor Lock-In
Many companies that want to improve GenAI integration across their tech ecosystem end up in one of two buckets:
- They get locked into a relationship with a hyperscaler or single vendor
- They haphazardly cobble together various component pieces like vector databases, embedding models, orchestration tools, and more.
Given how fast generative AI is changing, you don’t want to end up locked into either of these situations. You need to retain your optionality so you can quickly adapt as the tech needs of your business evolve or as the tech market changes. My recommendation? Use a flexible API system.
DataRobot can help you integrate with all of the major players, yes, but what’s even better is how we’ve built our platform to be agnostic about your existing tech and fit in where you need us to. Our flexible API provides the functionality and flexibility you need to actually unify your GenAI efforts across the existing tech ecosystem you’ve built.
2. Build Integration-Agnostic Models
In the same vein as avoiding vendor lock-in, don’t build AI models that only integrate with a single application. For instance, let’s say you build an application for Slack, but now you want it to work with Gmail. You might have to rebuild the entire thing.
Instead, aim to build models that can integrate with multiple different platforms, so you can be flexible for future use cases. This won’t just save you upfront development time. Platform-agnostic models will also lower your required maintenance time, thanks to fewer custom integrations that need to be managed.
With the right intelligence layer in place, you can bring the power of GenAI models to a diverse blend of apps and their users. This lets you maximize the investments you’ve made across your entire ecosystem. In addition, you’ll also be able to deploy and manage hundreds of GenAI models from one location.
For example, DataRobot could integrate GenAI models that work smoothly across enterprise apps like Slack, Tableau, Salesforce, and Microsoft Teams.
3. Bring Generative And Predictive AI into One Unified Experience
Many companies struggle with generative AI chaos because their generative and predictive models are scattered and siloed. For seamless integration, you need your AI models in a single repository, no matter who built them or where they’re hosted.
DataRobot is perfect for this; so much of our product’s value lies in our ability to unify AI intelligence across an organization, especially in partnership with hyperscalers. If you’ve built most of your AI frameworks with a hyperscaler, we’re just the layer you need on top to add rigor and specificity to your initiatives’ governance, monitoring, and observability.
And this isn’t just for generative or predictive models, but models built by anyone on any platform can be brought in for governance and operation right in DataRobot.
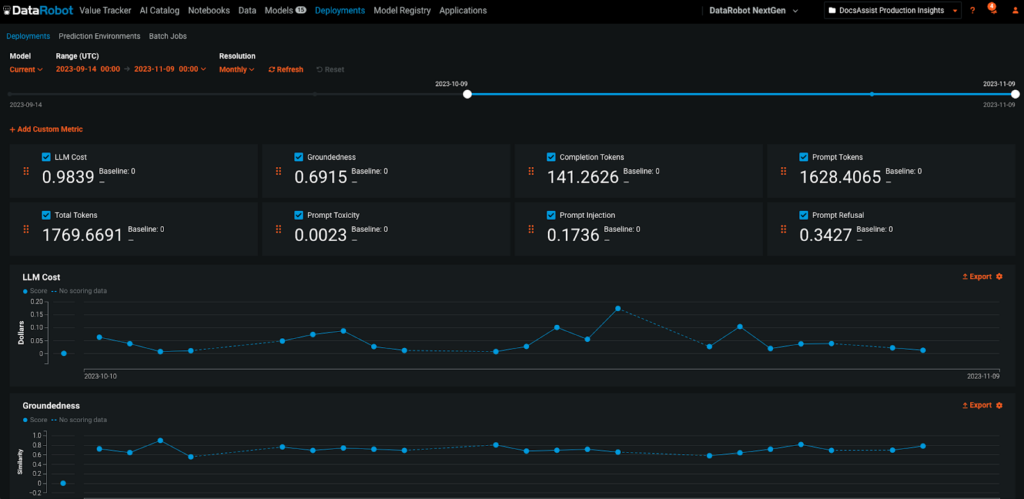
4. Build for Ease of Monitoring and Retraining
Given the pace of innovation with generative AI over the past year, many of the models I built six months ago are already out of date. But to keep my models relevant, I prioritize retraining, and not just for predictive AI models. GenAI can go stale, too, if the source documents or grounding data are out of date.
Imagine you have dozens of GenAI models in production. They could be deployed to all kinds of places such as Slack, customer-facing applications, or internal platforms. Sooner or later your model will need a refresh. If you only have 1-2 models, it may not be a huge concern now, but if you already have an inventory, it’ll take you a lot of manual time to scale the deployment updates.
Updates that don’t happen through scalable orchestration are stalling outcomes because of infrastructure complexity. This is especially critical when you start thinking a year or more down the road since GenAI updates usually require more maintenance than predictive AI.
DataRobot offers model version control with built-in testing to make sure a deployment will work with new platform versions that launch in the future. If an integration fails, you get an alert to notify you about the failure immediately. It also flags if a new dataset has additional features that aren’t the same as the ones in your currently deployed model. This empowers engineers and builders to be far more proactive about fixing things, rather than finding out a month (or further) down the line that an integration is broken.
In addition to model control, I use DataRobot to monitor metrics like data drift and groundedness to keep infrastructure costs in check. The simple truth is that if budgets are exceeded, projects get shut down. This can quickly snowball into a situation where whole teamsare affected because they can’t control costs. DataRobot allows me to track metrics that are relevant to each use case, so I can stay informed on the business KPIs that matter.
5. Stay Aligned With Business Leadership And Your End Users
The biggest mistake that I see AI practitioners make is not talking to people around the business enough. You need to bring in stakeholders early and talk to them often. This is not about having one conversation to ask business leadership if they’d be interested in a specific GenAI use case. You need to continuously affirm they still need the use case — and that whatever you’re working on still meets their evolving needs.
There are three components here:
- Engage Your AI Users
It’s crucial to secure buy-in from your end-users, not just leadership. Before you start to build a new model, talk to your prospective end-users and gauge their interest level. They’re the consumer, and they need to buy into what you’re creating, or it won’t get used. Hint: Make sure whatever GenAI models you build need to easily connect to the processes, solutions, and data infrastructures users are already in.
Since your end-users are the ones who’ll ultimately decide whether to act on the output from your model, you need to ensure they trust what you’ve built. Before or as part of the rollout, talk to them about what you’ve built, how it works, and most importantly, how it will help them accomplish their goals.
- Involve Your Business Stakeholders In The Development Process
Even after you’ve confirmed initial interest from leadership and end-users, it’s never a good idea to just head off and then come back months later with a finished product. Your stakeholders will almost certainly have a lot of questions and suggested changes. Be collaborative and build time for feedback into your projects. This helps you build an application that solves their need and helps them trust that it works how they want.
- Articulate Precisely What You’re Trying To Achieve
It’s not enough to have a goal like, “We want to integrate X platform with Y platform.” I’ve seen too many customers get hung up on short-term goals like these instead of taking a step back to think about overall goals. DataRobot provides enough flexibility that we may be able to develop a simplified overall architecture rather than fixating on a single point of integration. You need to be specific: “We want this Gen AI model that was built in DataRobot to pair with predictive AI and data from Salesforce. And the results need to be pushed into this object in this way.”
That way, you can all agree on the end goal, and easily define and measure the success of the project.
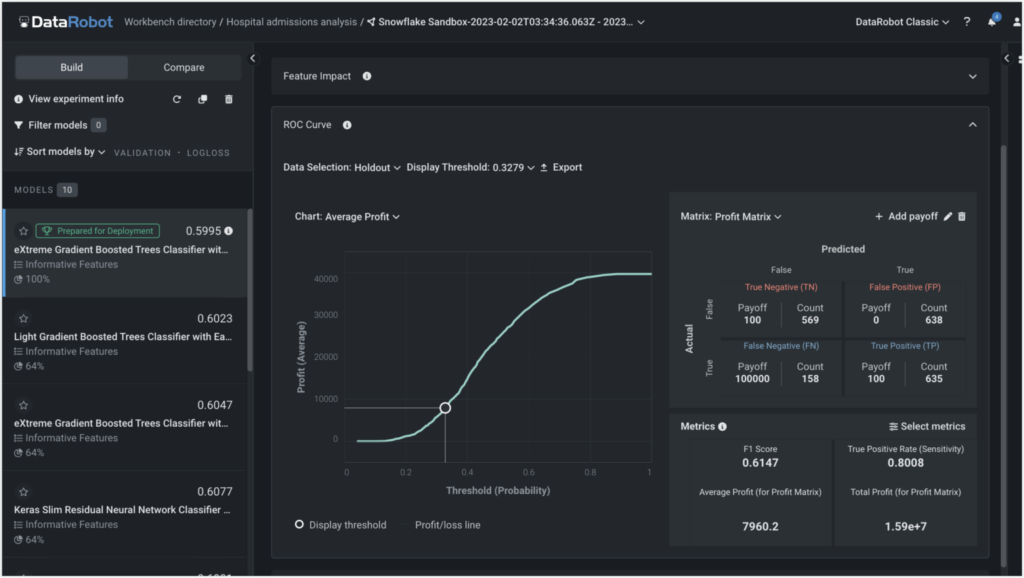
6. Move Beyond Experimentation To Generate Value Early
Teams can spend weeks building and deploying GenAI models, but if the process is not organized, all of the usual governance and infrastructure challenges will hamper time-to-value.
There’s no value in the experiment itself—the model needs to generate results (internally or externally). Otherwise, it’s just been a “fun project” that’s not producing ROI for the business. That is until it’s deployed.
DataRobot can help you operationalize models 83% faster, while saving 80% of the normal costs required. Our Playgrounds feature gives your team the creative space to compare LLM blueprints and determine the best fit.
Instead of making end-users wait for a final solution, or letting the competition get a head start, start with a minimum viable product (MVP).
Get a basic model into the hands of your end users and explain that this is a work in progress. Invite them to test, tinker, and experiment, then ask them for feedback.
An MVP offers two vital benefits:
- You can confirm that you’re moving in the right direction with what you’re building.
- Your end users get value from your generative AI efforts quickly.
While you may not provide a perfect user experience with your work-in-progress integration, you’ll find that your end-users will accept a bit of friction in the short term to experience the long-term value.
Unlock Seamless Generative AI Integration with DataRobot
If you’re struggling to integrate GenAI into your existing tech ecosystem, DataRobot is the solution you need. Instead of a jumble of siloed tools and AI assets, our AI platform could give you a unified AI landscape and save you some serious technical debt and hassle in the future. With DataRobot, you can integrate your AI tools with your existing tech investments, and choose from best-of-breed components. We’re here to help you:
- Avoid vendor lock-in and prevent AI asset sprawl
- Build integration-agnostic GenAI models that will stand the test of time
- Keep your AI models and integrations up to date with alerts and version control
- Combine your generative and predictive AI models built by anyone, on any platform, to see real business value
Ready to get more out of your AI with less friction? Get started today with a free 30-day trial or set up a demo with one of our AI experts.
Related posts
See other posts in Inside the ProductLearn how to build and scale agentic AI with NVIDIA and DataRobot. Streamline development, optimize workflows, and deploy AI faster with a production-ready AI stack.
What is an AI gateway? And why does your enterprise need one? Discover how it keeps Agentic AI scalable, secure, and cost-efficient. Read the full blog.
Explore syftr, an open source framework for discovering Pareto-optimal generative AI workflows. Learn how to optimize for accuracy, cost, and latency in real-world use cases.
Related posts
See other posts in Inside the ProductGet Started Today.