Explore the Most Popular Resources
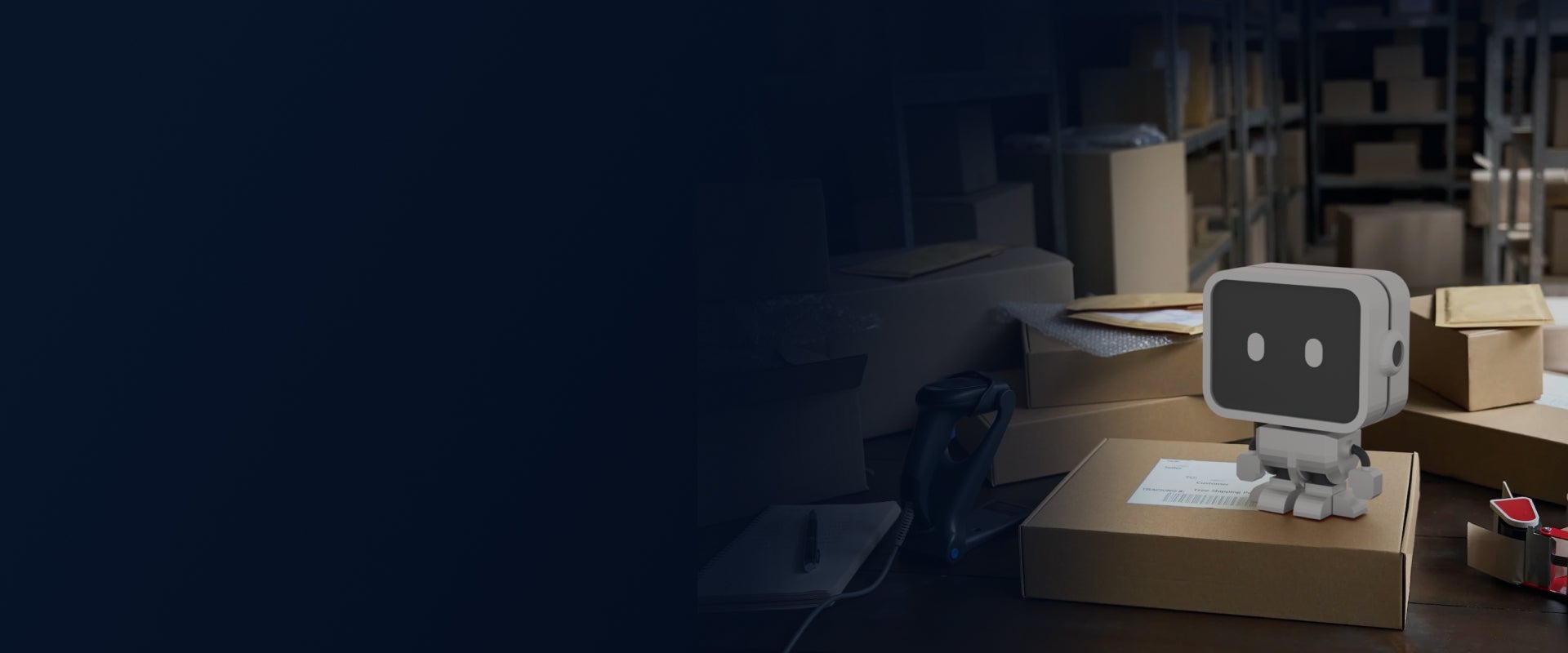
Ready to Get Started?
Take action now to transform your franchise organization with Ironside's data-driven decision-making solution.
Franchise customers with low AI maturity looking to be a more data-driven organization
Identify which behaviors to influence to optimize business performance and gain insights that are simultaneously developed across multiple features, variables, and may have complex interrelationships or dependencies.
Embrace targeted actions tailored to address specific challenges, departing from the generic one-size-fits-all approach. Drive your business forward with customized strategies for optimal results.
Establish an actionable and broadly applicable use for machine learning capability within the franchise organization, providing a foundation for developing and maturing data-driven decision-making.
Ironside’s Franchise Business Drivers Analysis identifies key performance factors influencing franchise store performance or revenue value of a customer. Using the most important driving factors, it additionally creates clusters or segments of similar stores or customers to enable targeted business actions. DataRobot is used for insights visualization and machine learning modeling.
Module 1: Identify which factors/features most drive a business outcome, such as:
Module 2: Based on the most important features discovered, perform a weighted clustering analysis to segment entities by drivers of the business outcome, such as:
This solution introduces a degree of supervision to the unsupervised method of clustering. Based on these clustering results, businesses can develop operational protocols that seek to influence only the most important drivers of business outcomes, ensuring the most efficient data-driven decision making.
Data wrangling
ML Model prediction and insights
Clustering
Ironside has extensive experience and expertise in franchise business model with multiple stores and/or a high volume of consumers. Ironside also possess strong machine learning and cloud technical talents to implement machine learning solutions. Here are some technical innovations by the team:
A major national hair salon franchise needed to understand why some of their store locations were performing well and some were having challenges. It was known that a variety of different factors and variables were potentially influencing store performance, but they needed to know the significance of each in order to prioritize operational improvement initiatives.
Ironside performed a business drivers analysis to examine factors including customer appointment no-shows, customer walk-in frequency, day of the week variations, holiday weekends, and more. The insights derived from this analysis led to specific plans to address the franchise labor scheduling practices, as well as salon-floor role management to improve store efficiency.
Ironside leverages the capabilities of DataRobot when developing a business drivers analysis, using advanced machine learning algorithms to get actionable insights from complex data. This solution measures and ranks the important factors driving outcomes as well as identifying categories or groups of revenue generating entities to enable targeted actions.
Take action now to transform your franchise organization with Ironside's data-driven decision-making solution.