Real world problems are multidimensional and multifaceted. Location data is a key dimension whose volume and availability has grown exponentially in the last decade. At the confluence of cloud computing, geospatial data analytics, and machine learning we are able to unlock new patterns and meaning within geospatial data structures that help improve business decision-making, performance, and operational efficiency.
The power of this convergence is demonstrated by the following example. Cleaned and enriched geospatial data combined with geostatistical feature engineering provides substantial positive impact on a housing price prediction model’s accuracy. The question we’ll be looking at is: What is the predicted sale price for a home sale listing? Keep in mind, however, that this workflow can be used for a broad range of geospatial use cases.
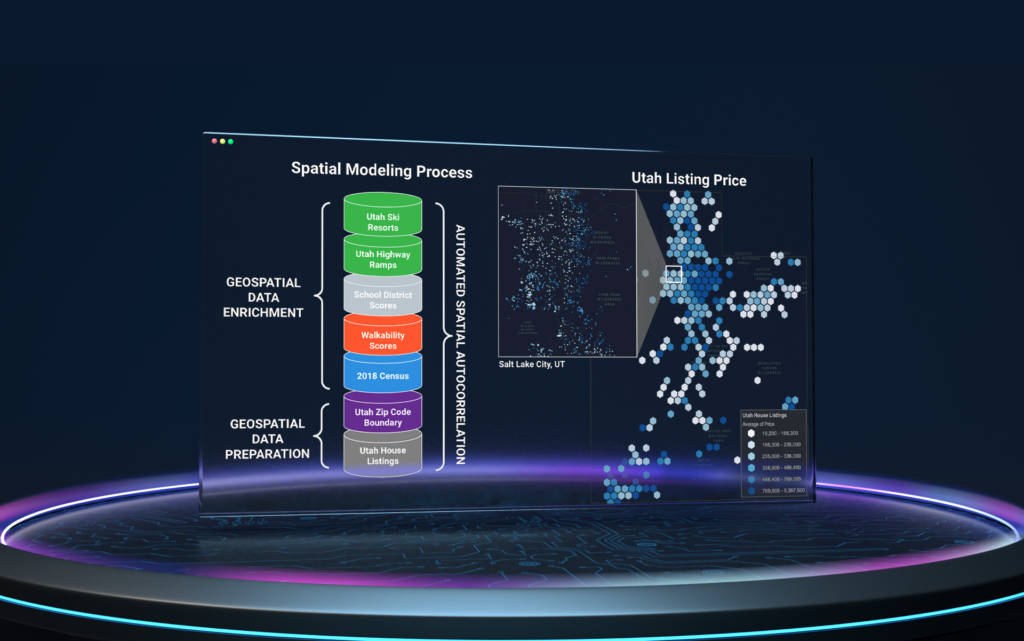
A Light Gradient Boosted Trees Regressor with Early Stopping model was trained without any geospatial data on 5,657 residential home listings to provide a baseline for comparison. This produced a RMSLE Cross Validation of 0.3530. By example, this model predicted a roughly $21,000 increase in price compared to its true price.
In order to isolate the impact of the geospatial features, we compare modeling results with the same blueprint as the baseline model using the data’s available location identifiers. Enabling spatial data in the modeling workflow resulted in a 7.14% RMSLE Cross Validation improvement from the baseline and a $12,000 increase in prediction price compared to the true price, roughly $9,000 lower than the baseline model.
As a practice, spatial data scientists attempt to transfer human-spatial reasoning for machines to learn from. Five hypothesized key factors that contribute to housing prices were used to enrich the listing data using spatial joins:
- select demographic variables from the U.S. Census Bureau,
- walkability scores from the Environmental Protection Agency,
- highway distance,
- school district scores, and
- distance to recreation, namely, ski resorts.
Geospatial enrichment in combination with Location AI’s Spatial Neighborhood Featurizer reveal local spatial dependence structures such as spatial autocorrelation that exists between number of bedrooms, the square footage of the listing data, and the enriched feature for walkability score. Spatial data enrichment resulted in a 8.73% RMSLE Cross Validation improvement from the baseline and a $1,300 increase in price compared to the true price, roughly $11,000 lower than the enabled dataset model and about $20,000 less than the baseline model.
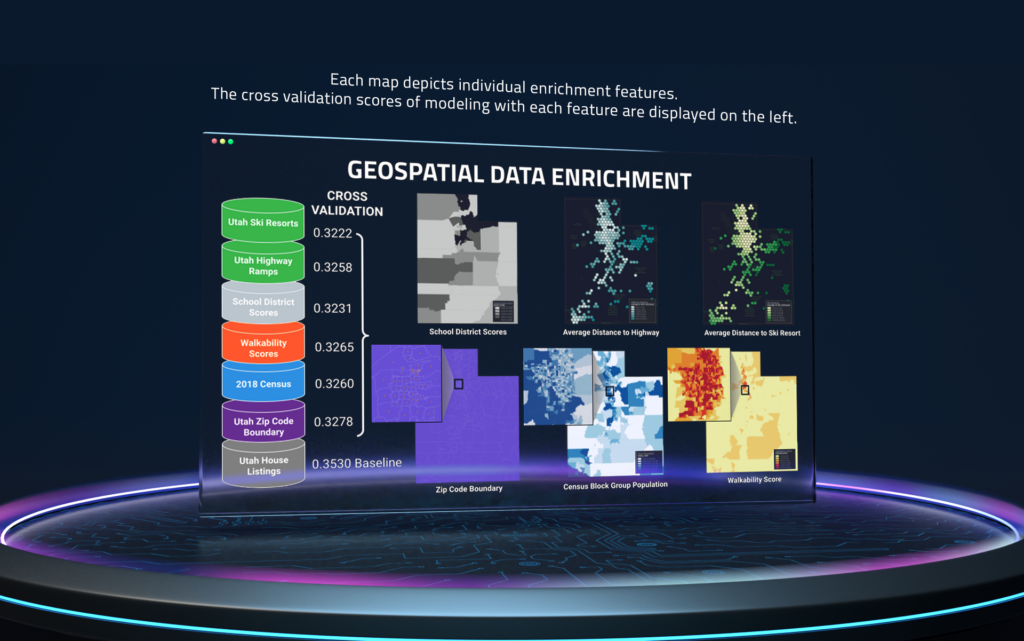
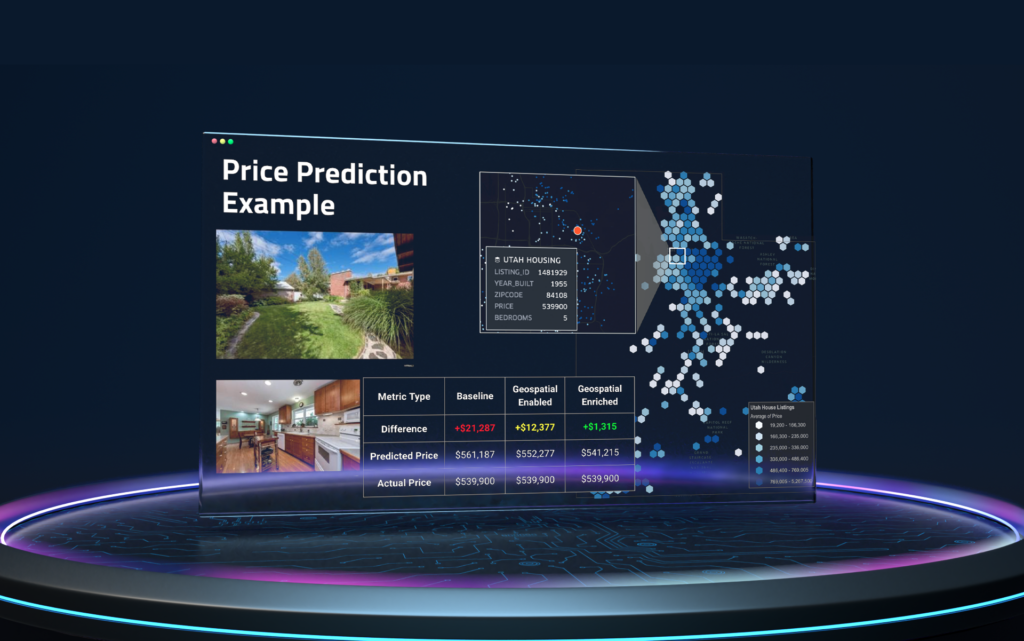
Spatial predictive modeling is applicable to a wide reach of industries because of the general availability of spatial data. Analyzing and understanding the applicability of spatial data enrichment to any particular machine learning scenario does not have to be a complex undertaking.
Learn how your team can develop, deliver, and govern AI apps and AI agents with DataRobot.
Request a DemoRelated posts
See other posts in AI for PractitionersAI adoption in supply chains isn’t about chasing trends. Learn the strategic framework that’s helping leaders build real, lasting impact. Read the full post.
Learn how to build and scale agentic AI with NVIDIA and DataRobot. Streamline development, optimize workflows, and deploy AI faster with a production-ready AI stack.
What is an AI gateway? And why does your enterprise need one? Discover how it keeps Agentic AI scalable, secure, and cost-efficient. Read the full blog.
Related posts
See other posts in AI for PractitionersGet Started Today.