Competition among businesses to acquire customer attention has never been higher. With digital marketing spend projected to reach $692.3B1 globally by 2024, companies should consider that more marketing does not necessarily lead to more customers acquisition. Companies offer incentives such as coupons to boost sales. By leveraging AI to target the right prospects with personalized promotions based on each customer’s unique attributes and purchase history, businesses can streamline customer segmentation and maximize conversions.
Initiate Robust Customer Engagement by Offering the Right Incentives
In a more traditional marketing approach, you’d take your customer list and segment it into distinct groups based on shared characteristics like region. You might then select a specific coupon for everyone in that segment to increase quarterly sales.
The trouble with this approach is that it often overlooks the unique desires and characteristics of individual customers. What might be the right incentive to buy for one customer may not entice a nearly identical customer. You need to find a more exact way to put the right material in front of each prospect to maximize engagement.
How Can AI Target the Right Prospects with Sharper Personalization?
Artificial intelligence (AI) can help improve the response rate on your coupon offers by letting you consider the unique characteristics and wide array of data collected online and offline of each customer and presenting them with the most attractive offers.
You may learn that customers who were grouped together using a traditional approach to market segmenting look very different after a machine learning assisted analysis.
To solve this problem, you can leverage datasets with demographic and transactional information along with product and marketing campaign details. Ingest your data and DataRobot will use all these data points to train a model—and once it is deployed, your marketing team will be able to get a prediction to know if a customer is likely to redeem a coupon or not and why.
All of this can be integrated with your marketing automation application of choice. For example, you could set up a data pipeline that delivers DataRobot predictions to HubSpot to automatically initiate offers within the business rules you set. You could also use the predictions to visualize a BI dashboard or report for your marketing managers to access.
From there, your marketing team can prioritize and target the clients that will receive coupons. DataRobot also gives you the details about how it came to that conclusion. This explainability of the predictions can help you see how and why the AI came to these predictions.
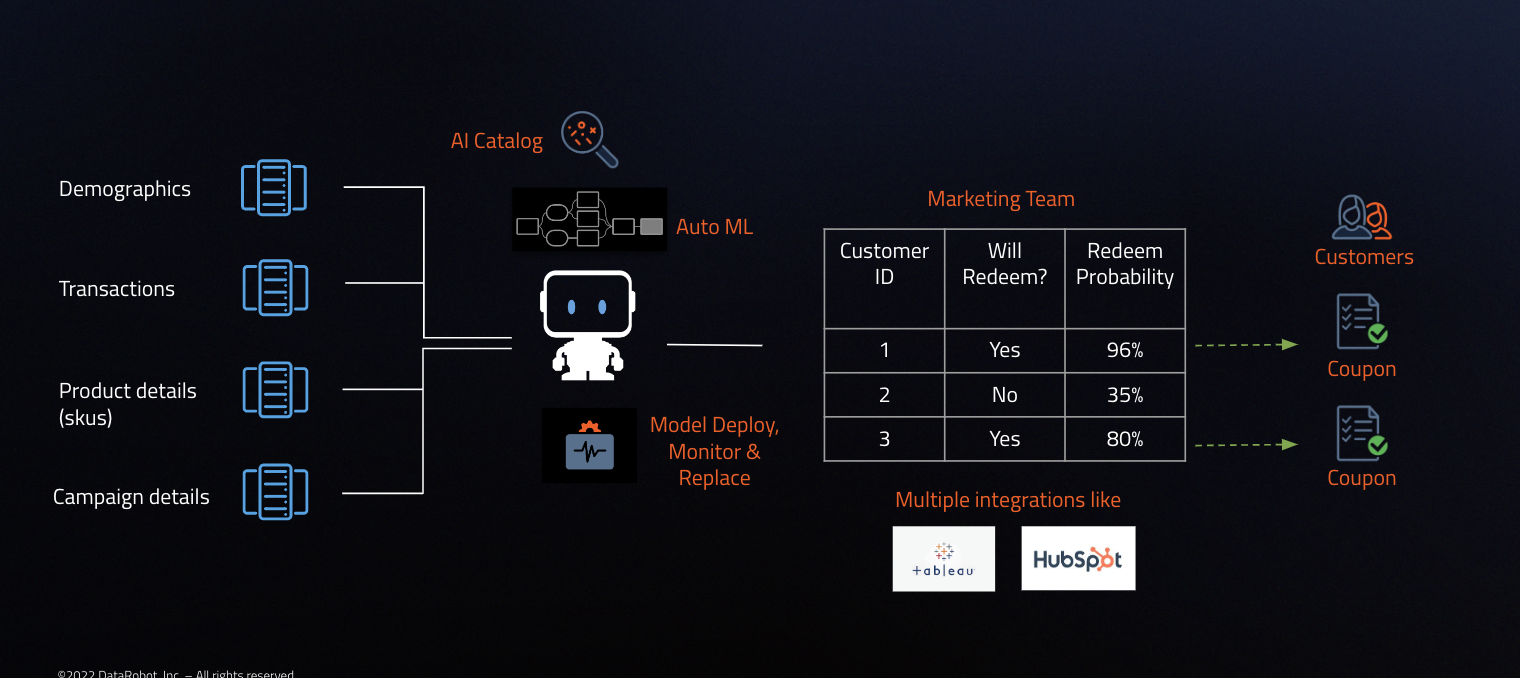
Get Started with DataRobot and Choose Your Target Variable
To get started with DataRobot, connect or import the datasets you already have from your existing mar-tech, CRM, and offline sales and marketing channels. You can upload all these datasets in our AI Catalog and start a project from there.
In this case, the datasets include demographic information from customers, plus a dataset with further information on the marketing campaigns, and two others that will provide information on past transactions and product information at the SKU level. All of these files have a combination of numeric, categorical, and date features, but remember that DataRobot can also handle images, text and location features.
I started my project with a simple data set with historical information of coupons sent to clients and a target variable that captured information about whether the coupon was redeemed or not in the past. As you upload your data, DataRobot will do some initial exploratory data analysis to get a deeper understanding of the dataset prior to model training. Next, choose your target variable—in this instance it is automatically detected as a classification problem and an optimization metric is recommended.
Automate Feature Engineering
DataRobot will accelerate machine learning by automating feature engineering, often considered one of the most laborious and time-consuming steps along the path to value. Traditional approaches are manual and require domain expertise. This means building hundreds of features for hundreds of machine learning algorithms—this approach to feature engineering is neither scalable nor cost-effective.
In contrast, DataRobot AI Platform simplifies the feature engineering process by automating the discovery and extraction of relevant explanatory variables from multiple related data sources. This allows you to build better machine learning models in less time and increase the pace of innovation with AI.
I started with a single dataset containing basic information on coupons redeemed or not by customers and enhanced it by joining additional secondary datasets from all the other relevant data sources. You can create a relationship configuration by using simple key joins or more complex multi-key joins between your datasets.
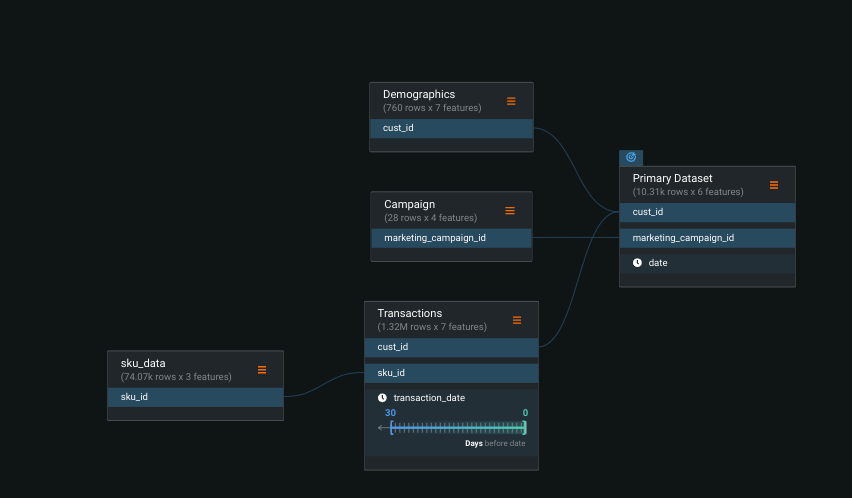
Training and Testing Different AI Models
As DataRobot starts building predictive models, a large repository of open source and proprietary packages will experiment with various modeling techniques. The model selection process will test several models to see which one is likely to yield the best results. Increase your workers count to build models in parallel with a large repository of open source and proprietary packages.
DataRobot will try out various modeling techniques and the models that will survive the first round will be fed more data and move on to the next round. Ultimately, only the best algorithms that solve specific problems will survive.
Looking at the model leaderboard, you can see that DataRobot built over 100 models and chose a winner. You can survey the model blueprint and see all of the pre-processing steps that were taken to get it ready.
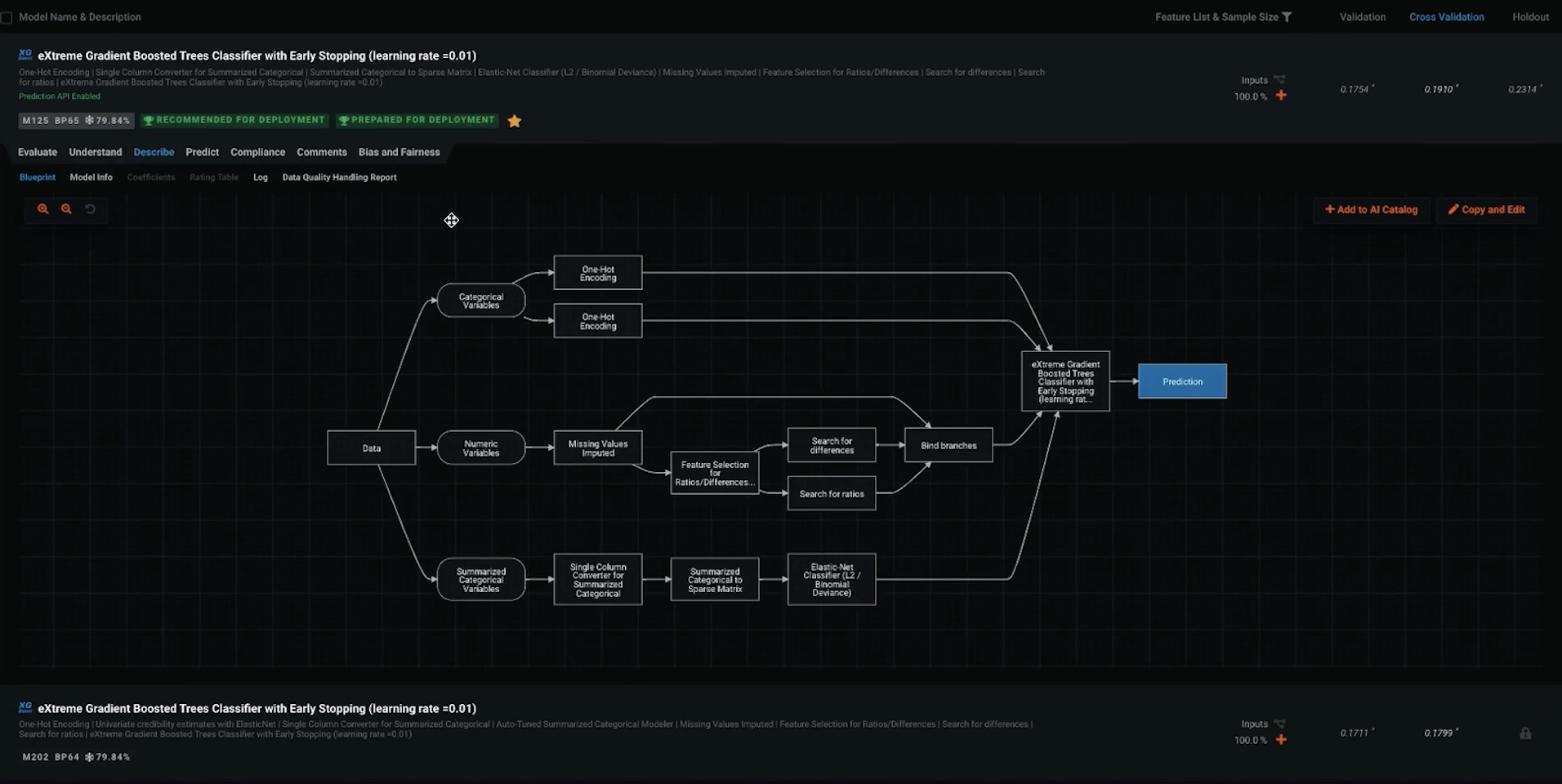
If you want more information, click on the links and DataRobot will generate clear documentation that explains the details of what DataRobot did within each particular step. Now, if you want to move forward with the model, the next step is to evaluate the fit.
Evaluate Model Fit and Understand How Features Are Impacting Predictions
The evaluation tab gives us some handy evaluation tools. The lift chart shows the fit of the model across the prediction distribution, while an ROC curve explores classification, performance, and statistics related to a selected model at any point on the probability scale.
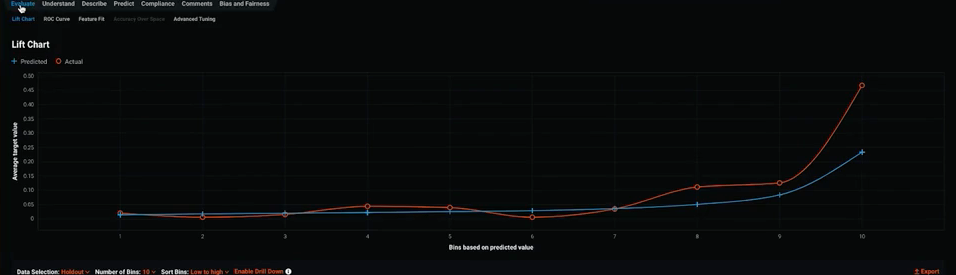
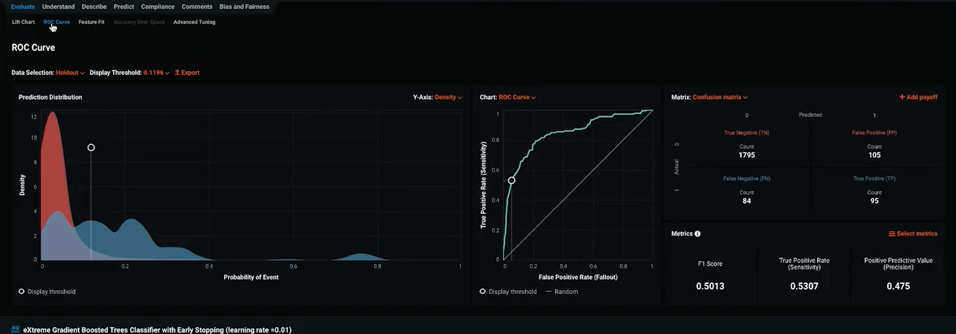
Once you’ve evaluated the fit of your model, the next step is to understand how the features are impacting predictions. Feature Discovery allows you to significantly improve the model’s overall performance by intelligently generating the right features for your models.
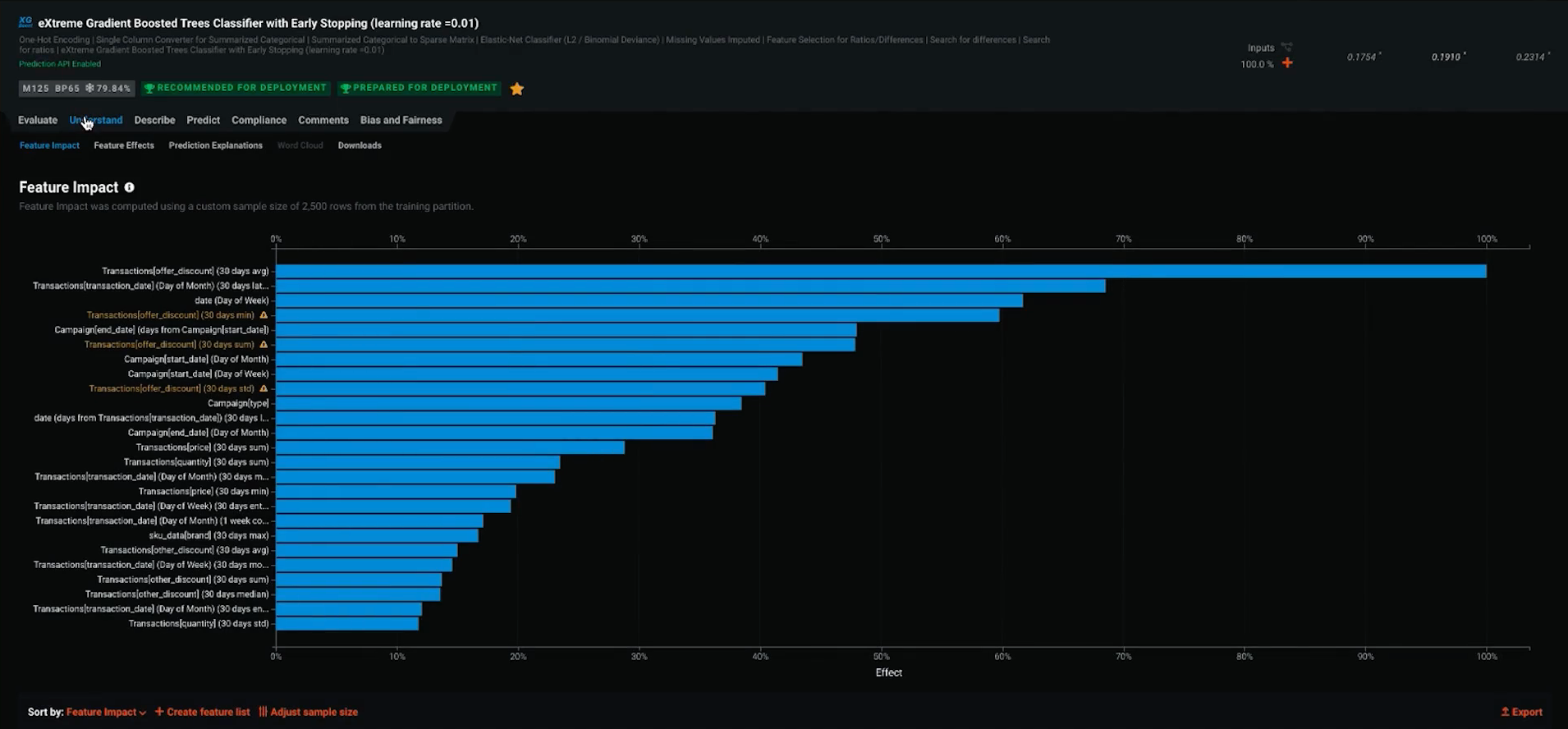
For this marketing offer model, the most important features are the average discount offer that a customer received in the last 30 days, the day of the month that a transaction takes place, the duration of a campaign, and other features with average sums and minimum values.
If you open these features, you can access feature lineage, which visualizes how a feature was created.
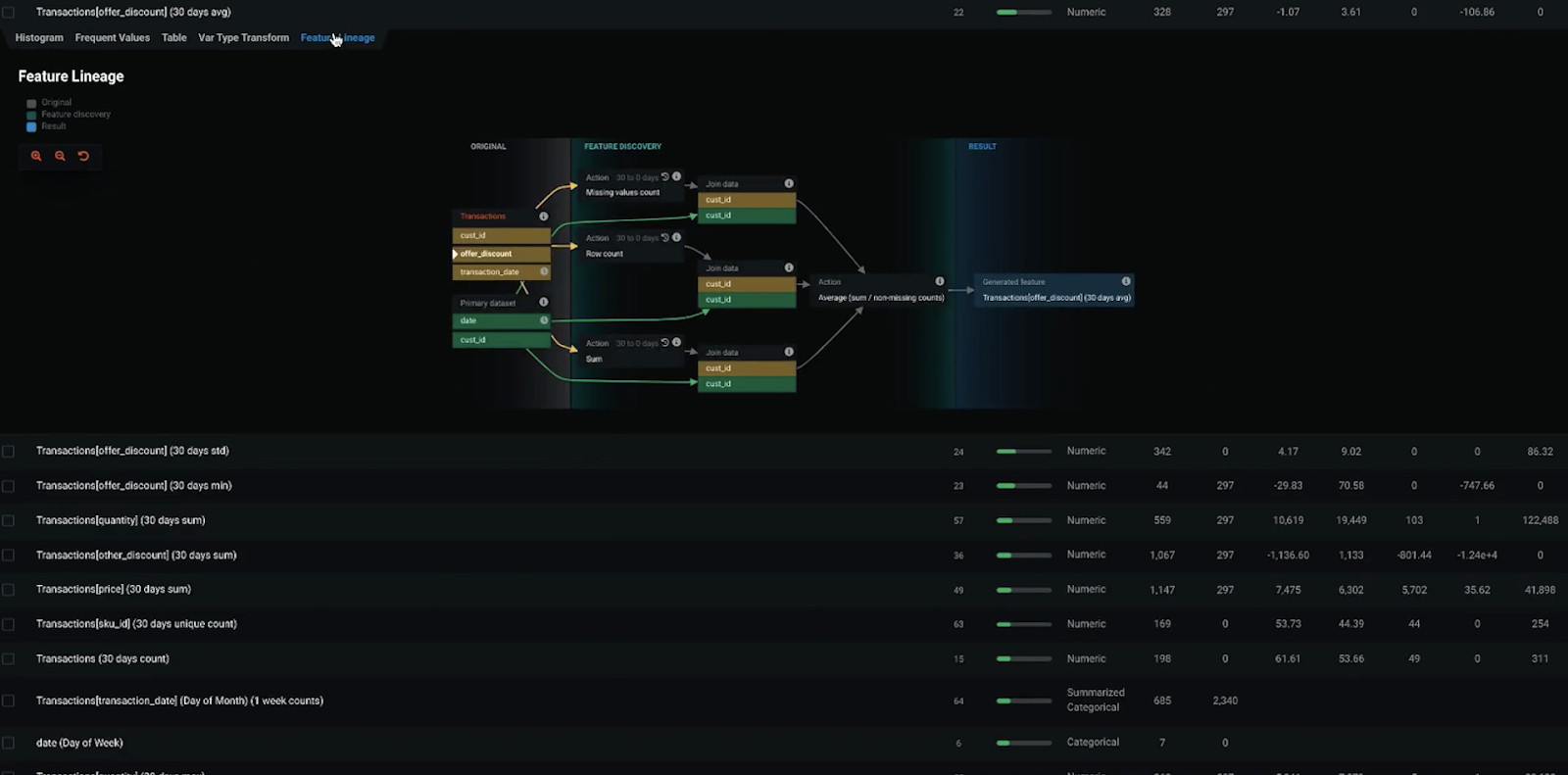
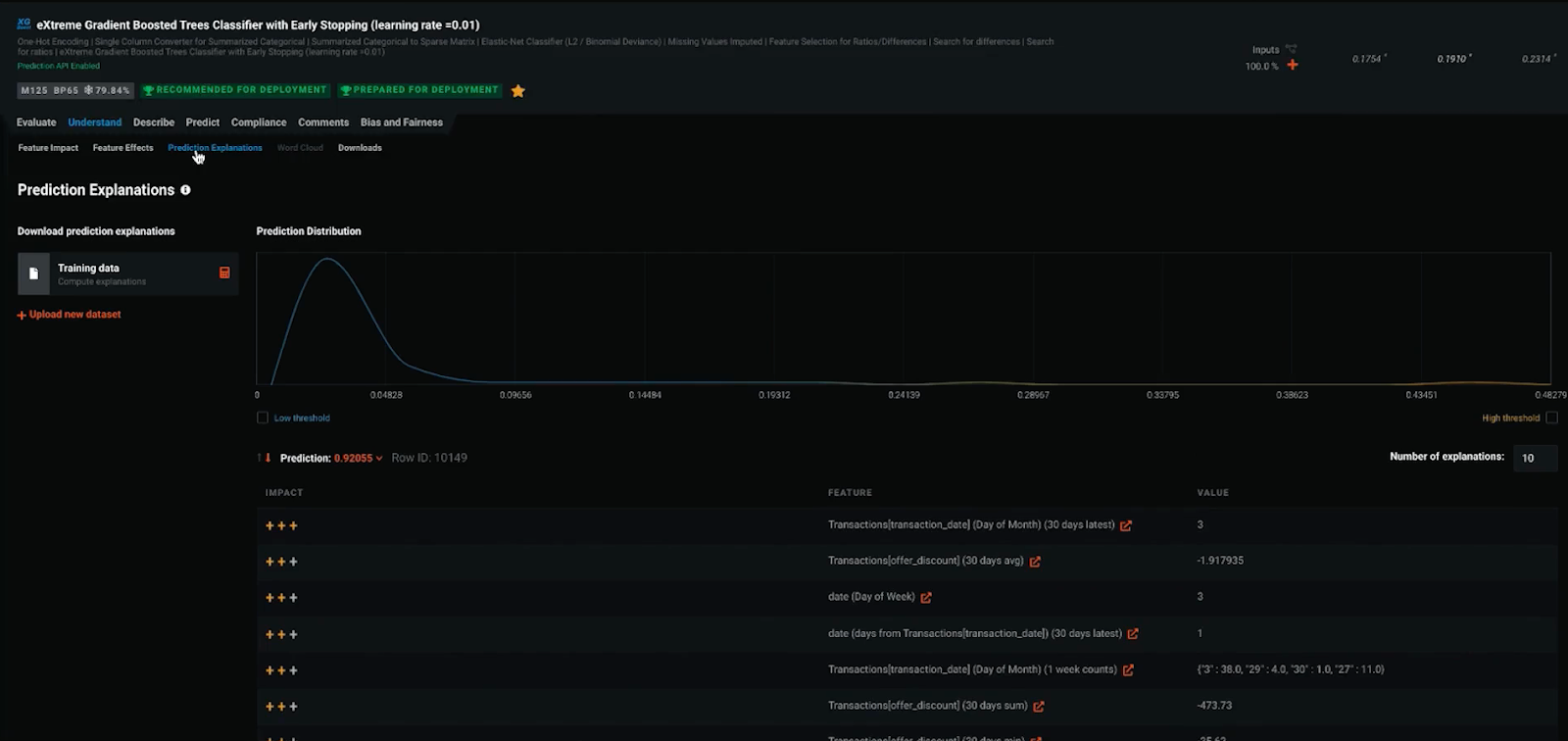
If the model looks good, it’s time to deploy it. DataRobot lets you deploy the model to an endpoint with an API that can serve up predictions in real time. If you click ‘Deployments’ you can see the DataRobot dashboard.
In this example, 17 active deployments are being monitored. By clicking on the Marketing Deployment, which has been serving predictions for a few days now, you can see an overview screen, which gives you:
- A top-line view on service health
- A look at data drift
- A clear picture of the model’s accuracy
You also have governance information, such as when and who created the deployment and who was involved in the review and approval workflow, which is important for audits and risk and compliance purposes.
Integrate Model Predictions with Your Existing Technology
After the model is in place and returning results, you can use a DataRobot API to integrate the model predictions with your existing mar-tech and CRM systems, like Tableau or HubSpot. This allows you to automate the process and offer targeted promotions to the specific customers who are most likely to use them.
To see how you can leverage AI to target your prospects and customers better with the promotions they’re most likely to accept, please request a demo.
Learn how your team can develop, deliver, and govern AI apps and AI agents with DataRobot.
Request a Demo1 https://www.statista.com/outlook/dmo/digital-advertising/worldwide#ad-spending
Related posts
See other posts in AI Across IndustriesLearn how to build and scale agentic AI with NVIDIA and DataRobot. Streamline development, optimize workflows, and deploy AI faster with a production-ready AI stack.
What is an AI gateway? And why does your enterprise need one? Discover how it keeps Agentic AI scalable, secure, and cost-efficient. Read the full blog.
Explore syftr, an open source framework for discovering Pareto-optimal generative AI workflows. Learn how to optimize for accuracy, cost, and latency in real-world use cases.
Related posts
See other posts in AI Across IndustriesGet Started Today.