Detect Fraudulent Credit Card Transactions
Overview
Fraudulent transactions are costly, but it is too expensive and inefficient to investigate every transaction for fraud. Even if possible, investigating innocent customers could prove to be a very poor customer experience, leading some clients to leave the business. Using AI, financial organizations can automate building an accurate model to predict the likelihood that a financial transaction is fraudulent. With the results you can create a rank-ordered queue of transactions for fraud units to investigate.
Business Problem
Credit card transaction fraud occurs when someone uses a credit card or credit account belonging to another person to make an unauthorized purchase. For instance, lost or stolen credit cards can be used to make in person or online purchases by people other than the card owners. Preventing fraud and keeping customers safe are key priorities. However, many existing fraud systems do not effectively prevent fraud since they leverage static rules that do not adapt to new fraudulent behavior and are time-consuming to update. In addition, since rules’ systems over-generalize specific constraints across varying transactions, they also lead to high numbers of false positives, where genuine transactions are incorrectly labeled as fraud. This disrupts customer experience during the transaction process.
Intelligent Solution
AI enhances fraud prevention systems by improving the accuracy of predicting which transactions are fraudulent. By holistically analyzing historical data from past fraudulent transactions, supervised machine learning models can be trained to identify fraud in future transactions.
In cases of new fraudulent behavior, unsupervised models, which do not require historically labeled data, will also flag anomalous transactions that show indications of potential fraud. These flagged transactions can be sent to manual reviewers for further investigations. Newly discovered fraudulent transactions can then be fed back to retrain the supervised models on an ongoing basis, allowing them to continuously adapt to the latest patterns and behaviors of fraud.
Overall, this means financial institutions will reduce the number of fraudulent transactions that go undetected, as well as avoid disruptions in their customers’ experiences by preventing genuine transactions from being flagged incorrectly.
Using DataRobot, you can automatically build extremely accurate predictive models to identify and prioritize likely fraudulent activity. Fraud units can then create a data-based queue, investigating only those incidents likely to require it. The resulting benefits are two-fold. First, your resources are deployed where you will see the greatest return on your investigative investment. Additionally, you optimize customer satisfaction by protecting their accounts and not challenging innocent transactions.
Technical Implementation
About the Data
For illustrative purposes, in this tutorial we use a simulated dataset that shows 10,000 transaction records from online merchants.
Problem Framing
The target variable for this use case is whether or not a transaction is fraudulent. This choice in target makes this a binary classification problem.
To create a model that can be trained to identify fraud, we need to feed it with data that can help it learn the characteristics of fraudulent transactions. Below are examples of the features that are important for this use case. Beyond these, we suggest incorporating additional data your organization collects which could also increase the accuracy of your model.
Information captured from a transaction:
- Payment Verification
- AVS Code, Payment Information
- Order Information
- Transaction amount, avg.transaction per item, # of items in order
Fraud signs in shipping:
- Shipping to alternate address
- Overnight shipping
- Is browser language translated
Customer Digital ID:
- Logged in to account or not
- Phone number
- Email domain information IP & Device info
Other Information:
- # of orders seen from same IP address over past 24 hours
- Customer service rep manual notes
Sample Feature List
Data Preparation
This dataset highlights common data elements which are usually captured during the end-to-end transaction process. This data is usually a good starting point for training the model. DataRobot can also process missing data, text data, and high-cardinality categorical data with various machine learning blueprints. You should be comfortable with creating new features out of the raw data based on domain expertise of specific fraud problems. Those engineered features can help to improve accuracy by separating the good behaviors of good customer transactions from the suspicious activities in fraudulent transactions.
10K rows of historical online transactions with fraud flag
Model Training
DataRobot Automated Machine Learning automates many parts of the modeling pipeline. Instead of hand-coding and manually testing dozens of models to find the one that best fits your needs, DataRobot automatically runs dozens of models and finds the most accurate one for you. In addition to training the models, DataRobot automates other steps in the modeling process such as processing and partitioning the dataset.
We will jump straight to interpreting the model results. Take a look here to see how to use DataRobot from start to finish and how to understand the data science methodologies embedded in its automation.
Interpret Results
DataRobot gives you transparency on how each model works to ensure you understand the drivers as to why transactions are labeled as fraudulent. This not only helps you improve your fraud prevention systems, but it also ensures you are aware of biases the model may have on race, age, etc.
Feature Impact
Feature Impact shows (on a macro level) the most important features when predicting fraudulent transactions. In the example below, we see the models take into account all features in the data to recognize fraud patterns. The features are sorted in descending order based upon the relative importance.
Feature Effects
Feature Effects shows the effect of changes in the value of each feature on the model’s predictions. It displays a graph depicting how a model “understands” the relationship between each feature and the target.
From these charts we make the following observations:
- Average Transaction Amount Per Item—The model reveals that fraudsters are more likely to target higher-value products.
- Email Domain—Fraudsters are more likely to use temporary emails to conduct fraud, whereas good customers will use their personal emails from common email service providers.
- Feature Effects—Whether Cookie ID is seen before. Newer Cookie IDs seen during a transaction indicate a higher risk of fraud.
Word Cloud
Word Cloud displays the most relevant words and short phrases that appear in unstructured text variables in a word cloud format. Text variables often contain words that are highly indicative of the response.
- Text strings are displayed in a color spectrum from blue to red, with blue indicating a negative effect and red indicating a positive effect.
- Text strings that appear more frequently are displayed in a larger font size, and those that appear less frequently are displayed in smaller font sizes.
In the fraud detection use case, we have collected the call center customer representative’s notes in free-form text format. They often contain information from manual reviews for the specific transactions. By leveraging DataRobot Automated Machine Learning, we can analyze every note available in the data and extract terms and features which can further improve the accuracy of the machine learning models.
Evaluate Accuracy
ROC Curve
The ROC Curve is a graphical means of illustrating classification performance for a model as the relevant performance statistics at all points on the probability scale change.
Confusion Matrix
The confusion matrix shows four possible outcomes of a classification problem.
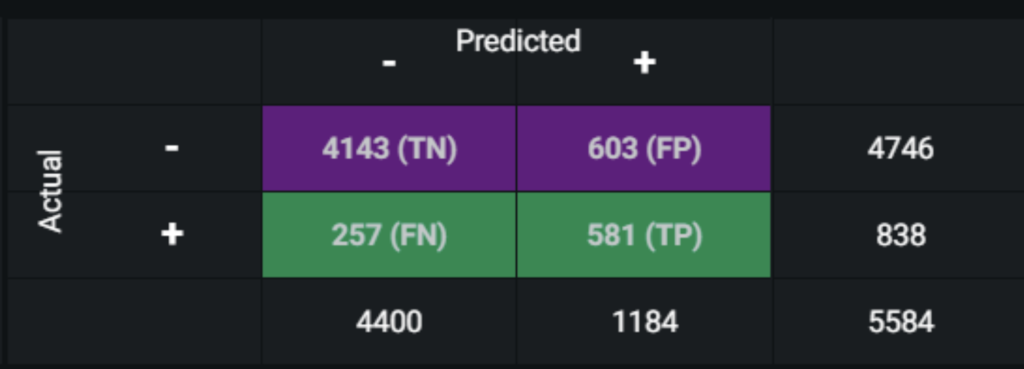
Post-Processing
Profit Curve—The question we face now is “How many false positives are acceptable and what is the cost of possibly missing fraudulent transactions?” This requires an assessment of incremental cost or profit associated with each outcome in the confusion matrix. The goal is to optimize the total profit and loss based on the selection of threshold. In DataRobot, we can provide inputs to different scenarios and assess the best decision strategies which will lead to an optimized ROI.
Business Implementation
Decision Environment
After you find the model that best learns patterns in your data to predict fraud likelihood, DataRobot makes it easy to deploy the model into your desired decision environment. Decision environments are the ways in which the predictions generated by the model will be consumed by the appropriate stakeholders in your organization and how they will ultimately make decisions using the predictions that impact your process.
This is a critical piece of implementing the use case as it ensures that predictions are used in the real world.
Decision Maturity
Automation | Augmentation | Blend
Fraud detection models can be deployed to make real-time decisions or supplement manual decisions. Either way, DataRobot provides corresponding model deployment mechanisms based on the speed and scale requirements of the use cases.
Model Deployment
DataRobot helps provide your fraud managers and fraud operations team with the information they need to capture signals of fraud in the transaction data. Companies can leverage the Prediction API to integrate them into their real-time decision engines and manual decision-making processes.
Decision Stakeholders
Decision Executors
Real-time decision engine + fraud ops case management
Decision Managers
Fraud Risk Manager, Finance P&L planner, Product Manager
Decision Authors
Data Scientist, Fraud Risk Manager
Decision Process
Real-Time Decisions
Real-time decisions can be made to approve or deny instances in processes such as credit card transactions, credit card applications, and online account login requests.
Once the data is captured, it can be aggregated and sent to DataRobot’s REST API deployment endpoint. The predictions are returned to notify the merchant / customer on a decision to automatically approve or decline the transaction, or ask for additional verification via OTP (one-time-password), KYC (know your customer) or additional security layer (e.g., 3D Secure), and so forth.
Manual Decisions
The results of the real-time decisions can also be visualized for the fraud operations team on a BI dashboard or report. This allows them to analyze the feature impact and feature effects of their fraud models to identify levers in the transaction process they can adjust to improve their overall fraud system. Under this notion, the new insights they capture from the AI models can also supplement any additional rules they embed into their rules systems if there are any significant features.
The BI dashboard or report can also visualize the results of unsupervised machine learning models, which inform the fraud operations team of anomalous patterns in their transactions that may shed light on new and unknown behaviors of fraud.
Model Monitoring
Models should be closely be monitored via DataRobot MLOps (and its model management and monitoring capabilities).
Service Health
- System downtime alert.
- Spike of data error due to schema mismatch.
- Prediction latency increase for real-time predictions.
Data Drift
- 3rd party data feed quality, providing real-time monitoring.
- Occurs when the patterns of the most important features (top%) are changing when compared to the training data
- The decision ratio is drifting, which may signal potential model decay or potential fraud spikes.
Accuracy
- If the model accuracy metric deteriorates, the model needs to be retrained with more recent data; replacing existing models with new models helps ensure model peak performance.
- Customer fraud disputes that are not captured by the model should be fed to retraining the model so that it will learn these patterns and embed them into the model’s decision making (False Negatives).
- Customer complaints on transaction friction will help inform the model of the false alarms it is causing (False Positives).
Implementation Risks
Model risks
- Failure to incorporate or engineer relevant features which may be helpful in capturing the behaviors of fraudulent transactions.
- Failure to perform out-of-time validation to assess the model’s performance over different vintages of data.
- Failure to remove target leakage from training data, which will result in inflated model performance.
- Failure to provide transparency of the model to ensure no bias was included during the model training phase.
- Failure to miss newly emergent patterns of fraud unseen in the training data.
Process risks
- Failure to include and convince all necessary stakeholders, e.g., marketing, product, IT, finance.
- Failure to build requirements and execute model integration for real-time predictions.
People risks
- Failure to design a proper customer service process to handle potential false positives and customer disruptions, which may lead to headline risk or reputational risk to the financial institutions who implemented machine learning solutions.
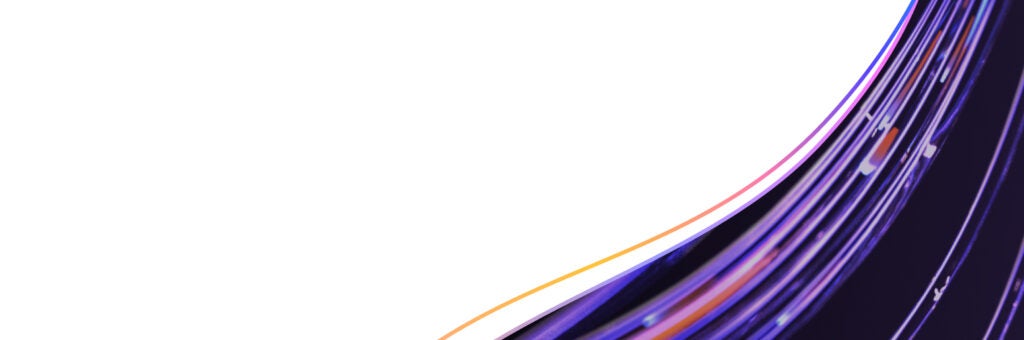
Experience the DataRobot AI Platform
Less Friction, More AI. Get Started Today With a Free 30-Day Trial.
Sign Up for FreeExplore More Use Cases
-
BankingBlockchain
As a relatively new financial system, blockchain is particularly vulnerable to security threats. Build and deploy machine learning algorithms that can detect anomalous behaviour anywhere along the chain.
Learn More -
BankingDigital Wealth Management
Use the power of AI to reach and engage new clients, matching the right customer with the right product.
Learn More -
BankingPredict Likelihood of Loan Default and Credit Default Rates
Making accurate judgments on the likelihood of default is the difference between a successful and unsuccessful loan portfolio. Reduce defaults and minimize risk by predicting the likelihood that a borrower will not repay their loan.
Learn More
-
BankingBlockchain
As a relatively new financial system, blockchain is particularly vulnerable to security threats. Build and deploy machine learning algorithms that can detect anomalous behaviour anywhere along the chain.
Learn More -
BankingDigital Wealth Management
Use the power of AI to reach and engage new clients, matching the right customer with the right product.
Learn More -
BankingPredict Likelihood of Loan Default and Credit Default Rates
Making accurate judgments on the likelihood of default is the difference between a successful and unsuccessful loan portfolio. Reduce defaults and minimize risk by predicting the likelihood that a borrower will not repay their loan.
Learn More