Releases
DataRobot 6.1
In Release 6.1, we focus on the business end of enterprise AI with a new use case value tracker. We’ve also introduced spatially aware models with Location AI, anomaly detection for Automated Time Series, and champion/challenger models for MLOps. Here are just some of the highlights.
DataRobot Location AI
Where Predictions Count. In DataRobot Release 6.1, we introduce spatial awareness to our Automated Machine Learning product. Location AI is a new patent pending feature that allows your predictive models to understand spatial relationships between observations in a dataset. For example, one of the best ways to predict home prices is to know the prices of other homes in the same neighborhood. Knowing the effects of proximity is critical for many prediction problems, and Location AI automates complex spatial modeling tasks for both novice and expert users. You can also mix your location data with other data types, including tabular, text, and images.
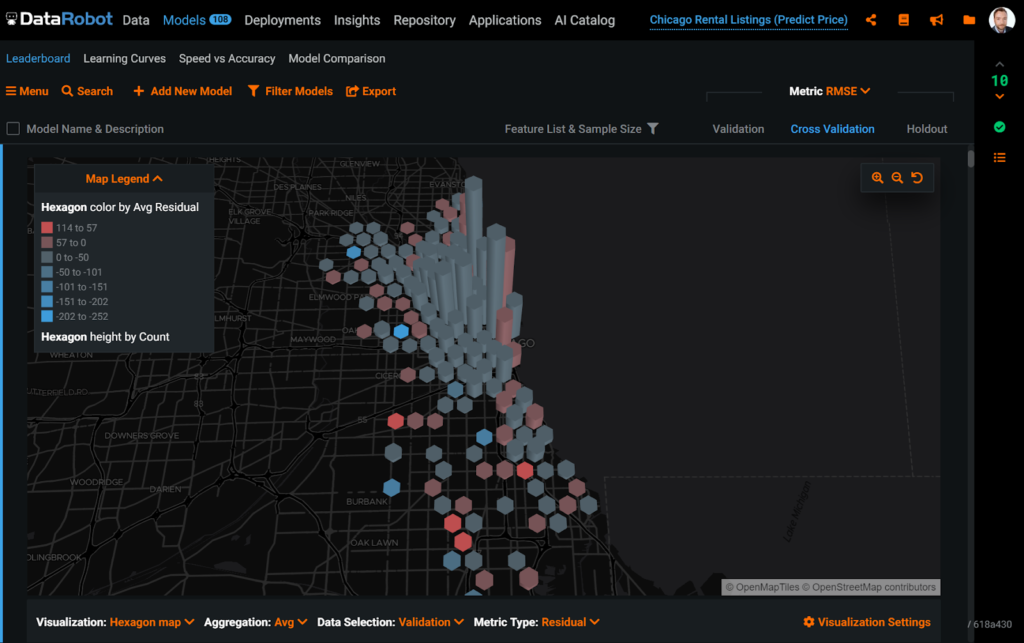
Use Case Value Tracker
Understand the ROI of Your AI. We are constantly adding features to help with the business operationalization of your machine learning efforts. In Release 6.1, the Use Case Value Tracker is our latest addition. It is a centralized hub to collaborate with team members around a single AI initiative end-to-end . Use Case Value Tracker enables you to manage your machine learning projects and understand the associated value at every step, organizing all your DataRobot assets around a given use case.
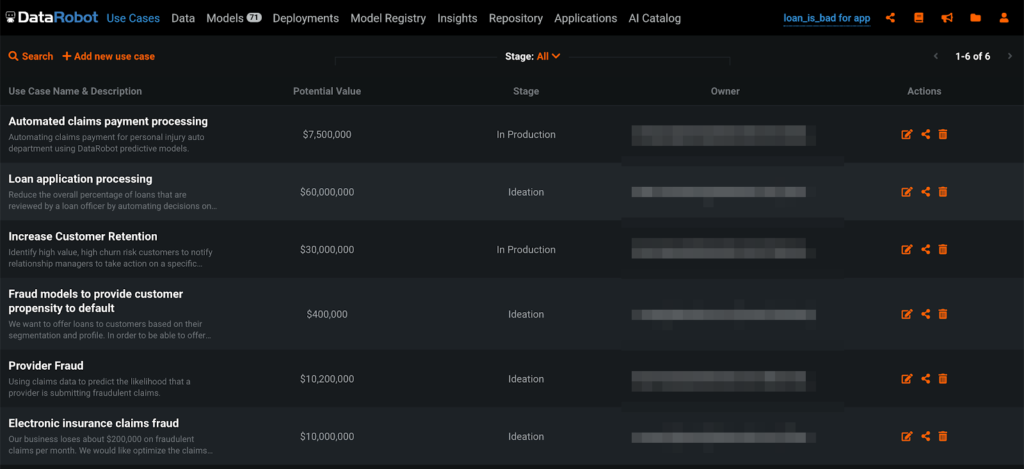
Profit Curves and Payoff Matrices
Know the Value of Your Predictions. Most organizations that are serious about AI need to be able to assess the financial impact of the predictions their models generate. In DataRobot 6.1, we introduce Profit Curve and Payoff Matrix so you can visualize the payoff from good predictions and the costs associated with bad predictions. You can set up a number of alternative payoff matrices, inspect the resulting profit curve, and even compare alternative profit curves on the same chart. You’ll be able to fine-tune your models for maximum business impact.
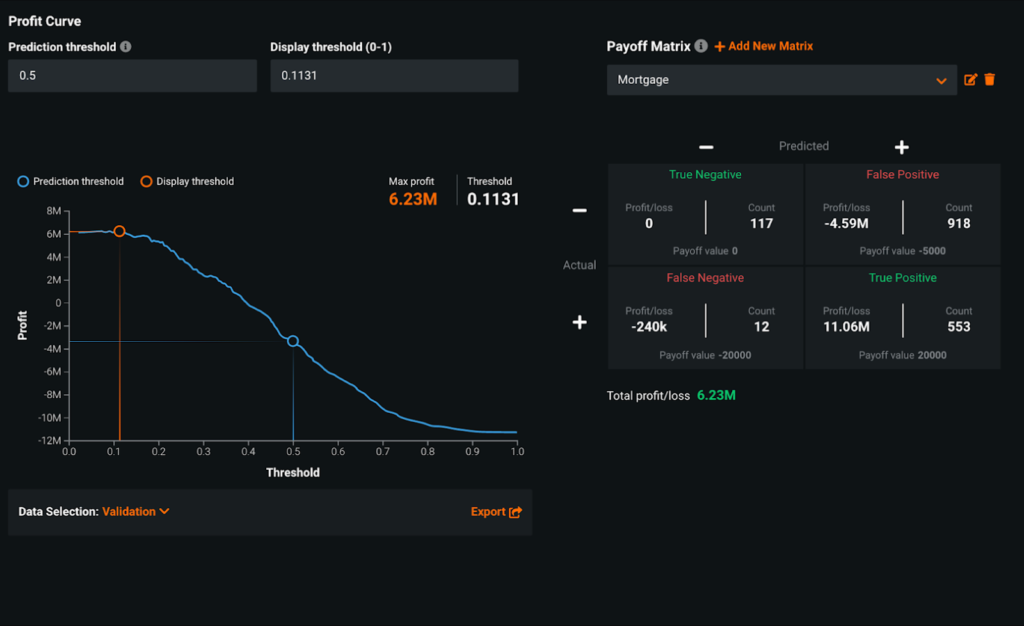
MLOps Champion/Challenger Models
When Production Models Compete. Accurate models today may not be accurate tomorrow. Champion/challenger models in MLOps Release 6.1 enable you to test and compare your production models with alternatives as business conditions change. This allows you to continue the same rigorous analysis of your production models as you did during training. You can replay predictions against challenger models to analyze accuracy and performance over the same time period. When a challenger beats out the current champion, you can instantly switch out your old model with absolutely no service interruption.
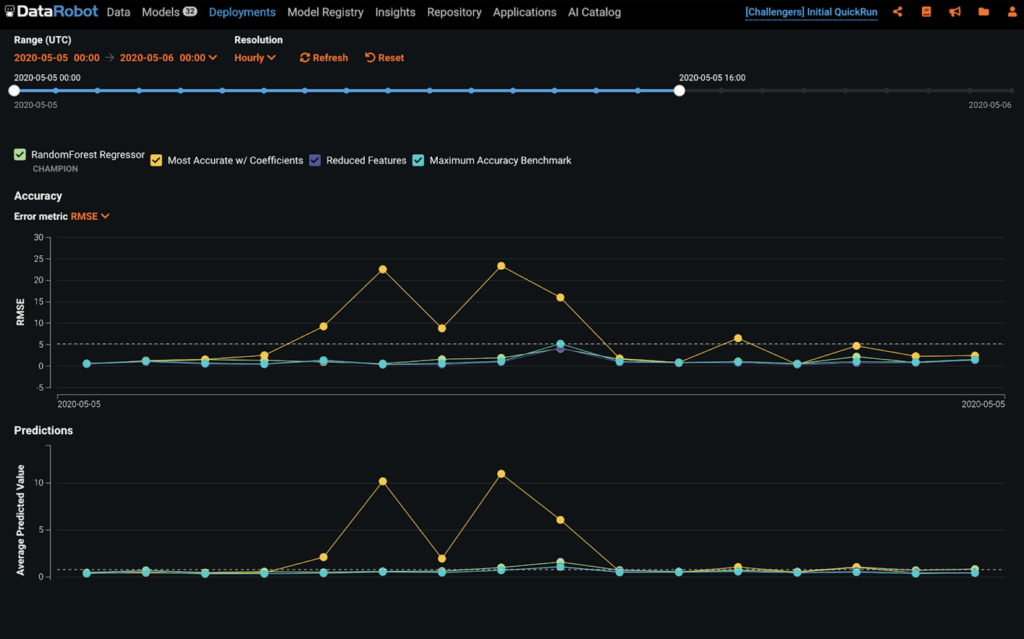
Automated Time Series Anomaly Detection
Expect the Unexpected. In this release, we introduce Automated Time Series Anomaly Detection. Without the need to specify a target variable, you can now train time series models in a fully unsupervised mode. DataRobot automatically selects, builds, tests, and ranks a diverse set of anomaly detection models and uses a novel Synthetic AUC error metric to help you understand which model is best for your specific use case. DataRobot’s Automated Time Series Anomaly Detection also provides all of the automated insights and visualizations to help you explain every model and drill into individual anomalies to understand causal factors. Anomaly Detection unlocks a wide variety of new use cases for Automated Time Series customers.
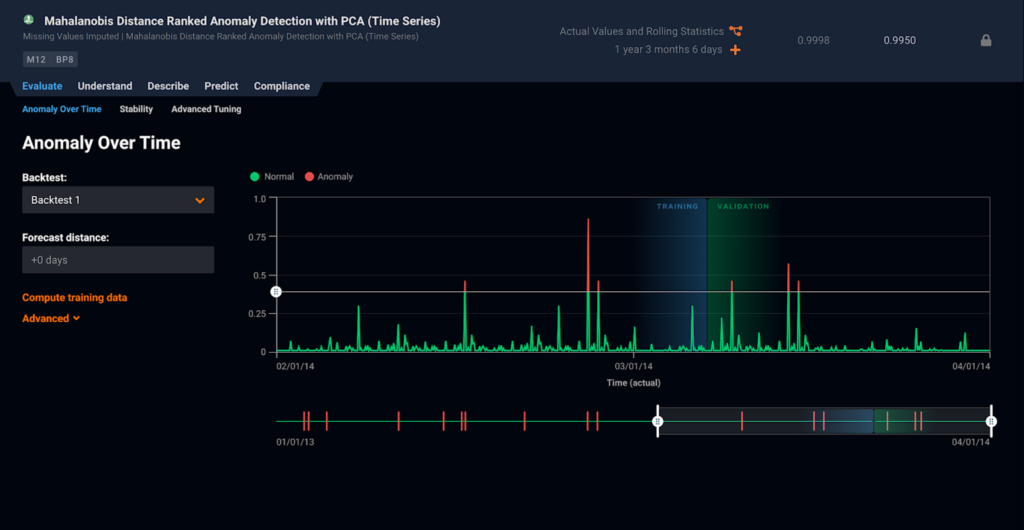
More for AI Explainability and Trust
It’s not hard to be humble. With every release, DataRobot aims to bring more features to help you explain and trust your AI. Release 6.1 is no exception. We have introduced an automated Data Quality Assessment and Data Quality Handling report that surfaces and reports on issues, such as missing values, outliers, target leakage, and more. We now provide intuitive SHAP explanations to show you exactly to what extent each feature is responsible for predictions that deviate from the average. In addition, a unique Humble AI feature in MLOps allows you to define rules and take corrective action when a production model makes an uncertain prediction.
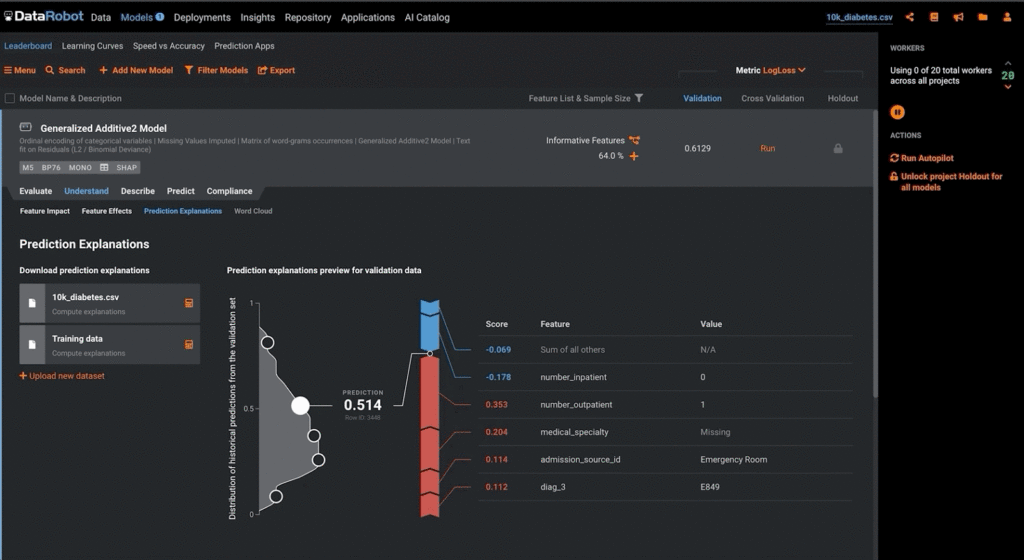