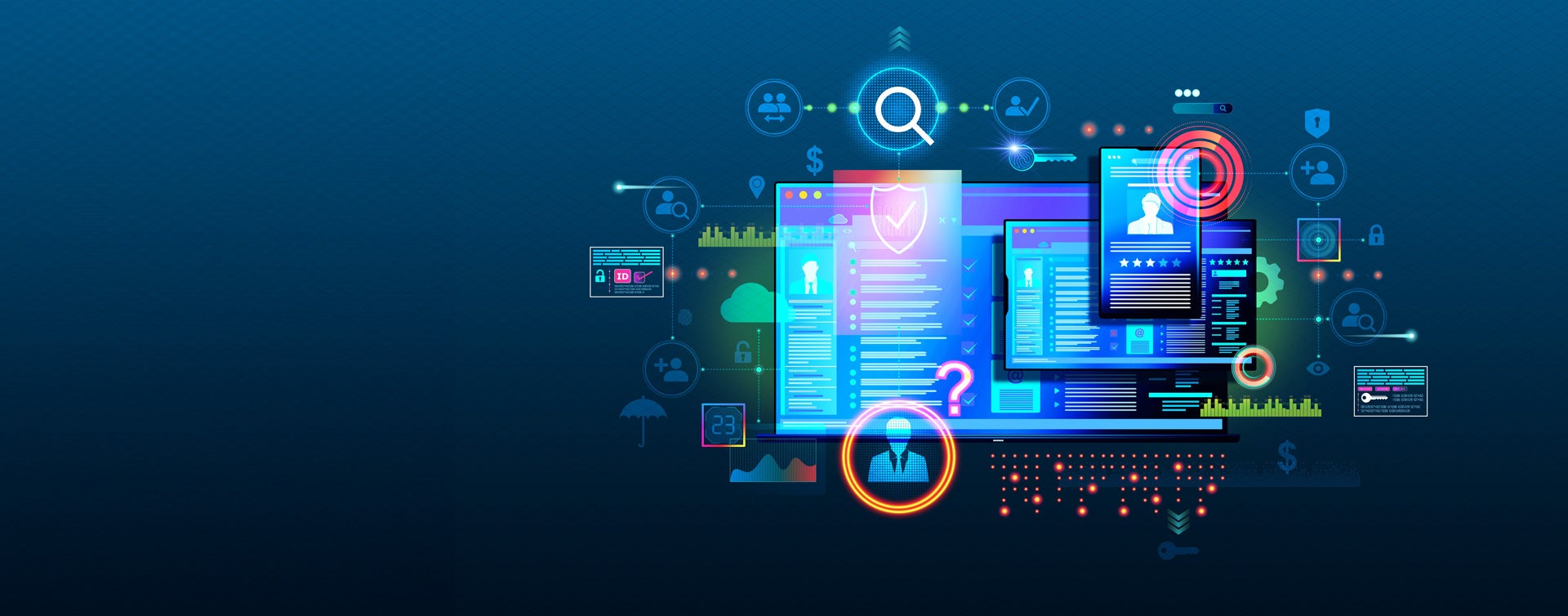
- Blog
- Generative AI
- Potential Risks of Generative AI According to NAIAC – And How to Mitigate Them
Potential Risks of Generative AI According to NAIAC – And How to Mitigate Them
The unprecedented rise of Artificial Intelligence (AI) has brought transformative possibilities across various sectors, from industries and economies to societies at large. However, this technological leap also introduces a set of potential challenges. In its recent public meeting, the National AI Advisory Committee (NAIAC)1, which provides recommendations on topics including the current state of the U.S. AI competitiveness, the state of science around AI, and AI workforce issues to the President and the National AI Initiative Office, has voted on a finding based on expert briefing on the potential risks of AI and more specifically generative AI2. This blog post aims to shed light on these concerns and delineate how DataRobot customers can proactively leverage the platform to mitigate these threats.
Understanding AI’s Potential Risks
With the swift rise of AI in the realm of technology, it stands poised to transform sectors, streamline operations, and amplify human potential. Yet, these unmatched progressions also usher in a myriad of challenges that demand attention. The “Findings on The Potential Future Risks of AI” discusses segments the risk of AI in short-term and long-term risks of AI. The near-term risks of AI, as described in the finding, refers to risks associated with AI that are well known and current concerns for AI, whether predictive or generative AI. On the other hand, long-term risks of AI underscores the potential risks of AI that may not materialize given the current state of AI technology or well understood but we should prepare for their potential impacts. This finding highlights a few categories of AI risks – malicious objective or unintended consequences, economic and societal, and catastrophic.
Societal
While Large Language Models (LLMs) are primarily optimized for text prediction tasks, their broader applications don’t adhere to a singular goal. This flexibility allows them to be employed in content creation for marketing, translation, or even in disseminating misinformation on a large scale. In some instances, even when the AI’s objective is well-defined and tailored for a specific purpose, unforeseen negative outcomes can still emerge. In addition, as AI systems evolve in complexity, there’s a growing concern that they might find ways to circumvent the safeguards established to monitor or restrict their behavior. This is especially troubling since, although humans create these safety mechanisms with particular goals in mind, an AI may perceive them differently or pinpoint vulnerabilities.
Economic
As AI and automation sweep across various sectors, they promise both opportunities and challenges for employment. While there’s potential for job enhancement and broader accessibility by leveraging generative AI, there’s also a risk of deepening economic disparities. Industries centered around routine activities might face job disruptions, yet AI-driven businesses could unintentionally widen the economic divide. It’s important to highlight that being exposed to AI doesn’t directly equate to job loss, as new job opportunities may emerge and some workers might see improved performance through AI support. However, without strategic measures in place—like monitoring labor trends, offering educational reskilling, and establishing policies like wage insurance—the specter of growing inequality looms, even if productivity soars. But the implications of this shift aren’t merely financial. Ethical and societal issues are taking center stage. Concerns about personal privacy, copyright breaches, and our increasing reliance on these tools are more pronounced than ever.
Catastrophic
The evolving landscape of AI technologies has the potential to reach more advanced levels. Especially, with the adoption of generative AI at scale, there’s growing apprehension about their disruptive potential. These disruptions can endanger democracy, pose national security risks like cyberattacks or bioweapons, and instigate societal unrest, particularly through divisive AI-driven mechanisms on platforms like social media. While there’s debate about AI achieving superhuman prowess and the magnitude of these potential risks, it’s clear that many threats stem from AI’s malicious use, unintentional fallout, or escalating economic and societal concerns.
Recently, discussion on the catastrophic risks of AI has dominated the conversations on AI risk, especially with regards to generative AI. However, as was put forth by NAIAC, “Arguments about existential risk from AI should not detract from the necessity of addressing existing risks of AI. Nor should arguments about existential risk from AI crowd out the consideration of opportunities that benefit society.”3
The DataRobot Approach
The DataRobot AI Platform is an open, end-to-end AI lifecycle platform that streamlines/simplifies how you build, govern, and operate generative and predictive AI. Designed to unify your entire AI landscape, teams and workflows, it empowers you to deliver real-world value from your AI initiatives, while giving you the flexibility to evolve, and the enterprise control to scale with confidence.
DataRobot serves as a beacon in navigating these challenges. By championing transparent AI models through automated documentation during the experimentation and in production, DataRobot enables users to review and audit the building process of AI tools and its performance in production, which fosters trust and promotes responsible engagement. The platform’s agility ensures that users can swiftly adapt to the rapidly evolving AI landscape. With an emphasis on training and resource provision, DataRobot ensures users are well-equipped to understand and manage the nuances and risks associated with AI. At its core, the platform prioritizes AI safety, ensuring that responsible AI use is not just encouraged but integral from development to deployment.
With regards to generative AI, DataRobot has incorporated a trustworthy AI framework in our platform. The chart below highlights the high level view of this framework.
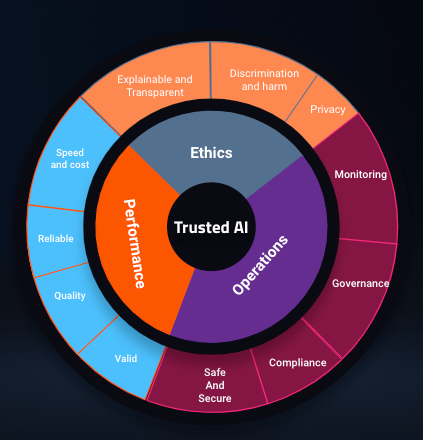
Pillars of this framework, Ethics, Performance, and Operations, have guided us to develop and embed features in the platform that assist users in addressing some of the risks associated with generative AI. Below we delve deeper into each of these components.
Ethics
AI Ethics pertains to how an AI system aligns with the values held by both its users and creators, as well as the real-world consequences of its operation. Within this context, DataRobot stands out as an industry leader by incorporating various features into its platform to address ethical concerns across three key domains: Explainability, Discrimination and harm mitigation, and Privacy preservation.
DataRobot directly tackles these concerns by offering cutting-edge features that monitor model bias and fairness, apply innovative prediction explanation algorithms, and implement a platform architecture designed to maximize data protection. Additionally, when orchestrating generative AI workflows, DataRobot goes a step further by supporting an ensemble of “guard” models. These guard models play a crucial role in safeguarding generative use cases. They can perform tasks such as topic analysis to ensure that generative models stay on topic, identify and mitigate bias, toxicity, and harm, and detect sensitive data patterns and identifiers that should not be utilized in workflows.
What’s particularly noteworthy is that these guard models can be seamlessly integrated into DataRobot’s modeling pipelines, providing an extra layer of protection around Language Model (LLM) workflows. This level of protection instills confidence in users and stakeholders regarding the deployment of AI systems. Furthermore, DataRobot’s robust governance capabilities enable continuous monitoring, governance, and updates for these guard models over time through an automated workflow. This ensures that ethical considerations remain at the forefront of AI system operations, aligning with the values of all stakeholders involved.
Performance
AI Performance pertains to evaluating how effectively a model accomplishes its intended goal. In the context of an LLM, this could involve tasks such as responding to user queries, summarizing or retrieving key information, translating text, or avarious other use-cases. It is worth noting that many existing LLM deployments often lack real-time assessment of validity, quality, reliability, and cost. DataRobot, however, has the capability to monitor and measure performance across all of these domains.
DataRobot’s distinctive blend of generative and predictive AI empowers users to create supervised models capable of assessing the correctness of LLMs based on user feedback. This results in the establishment of an LLM correctness score, enabling the evaluation of response effectiveness. Every LLM output is assigned a correctness score, offering users insights into the confidence level of the LLM and allowing for ongoing tracking through the DataRobot LLM Operations (LLMOps) dashboard. By leveraging domain-specific models for performance assessment, organizations can make informed decisions based on precise information.
DataRobot’s LLMOps offers comprehensive monitoring options within its dashboard, including speed and cost tracking. Performance metrics such as response and execution times are continuously monitored to ensure timely handling of user queries. Furthermore, the platform supports the use of custom metrics, enabling users to tailor their performance evaluations. For instance, users can define their own metrics or employ established measures like Flesch reading-ease to gauge the quality of LLM responses to inquiries. This functionality facilitates the ongoing assessment and improvement of LLM quality over time.
Operations
AI Operations focuses on ensuring ith the reliability of the system or the environment housing the AI technology. This encompasses not only the reliability of the core system but also the governance, oversight, maintenance, and utilization of that system, all with the overarching goal of ensuring efficient, effective, and safe and secure operations.
With over 1 million AI projects operationalized and delivering over 1 trillion predictions, the DataRobot platform has established itself as a robust enterprise foundation capable of supporting and monitoring a diverse array of AI use cases. The platform boasts built-in governance features that streamline development and maintenance processes. Users benefit from custom environments that facilitate the deployment of knowledge bases with pre-installed dependencies, expediting development lifecycles. Critical knowledge base deployment activities are logged meticulously to ensure that key events are captured and stored for reference. DataRobot seamlessly integrates with version control, promoting best practices through continuous integration/continuous deployment (CI/CD) and code maintenance. Approval workflows can be orchestrated to ensure that LLM systems undergo proper approval processes before reaching production. Additionally, notification policies keep users informed about key deployment-related activities.
Security and safety are paramount considerations. DataRobot employs two-factor authentication and access control mechanisms to ensure that only authorized developers and users can utilize LLMs.
DataRobot’s LLMOps monitoring extends across various dimensions. Service health metrics track the system’s ability to respond quickly and reliably to prediction requests. Crucial metrics like response time provide essential insights into the LLM’s capacity to address user queries promptly. Furthermore, DataRobot’s customizable metrics capability empowers users to define and monitor their own metrics, ensuring effective operations. These metrics could encompass overall cost, readability, user approval of responses, or any user-defined criteria. DataRobot’s text drift feature enables users to monitor changes in input queries over time, allowing organizations to analyze query changes for insights and intervene if they deviate from the intended use case. As organizational needs evolve, this text drift capability serves as a trigger for new development activities.
DataRobot’s LLM-agnostic approach offers users the flexibility to select the most suitable LLM based on their privacy requirements and data capture policies. This accommodates partners, which enforce enterprise privacy, as well as privately hosted LLMs where data capture is not a concern and is managed by the LLM owners. Additionally, it facilitates solutions where network egress can be controlled. Given the diverse range of applications for generative AI, operational requirements may necessitate various LLMs for different environments and tasks. Thus, an LLM-agnostic framework and operations are essential.
It’s worth highlighting that DataRobot is committed to continually enhancing its platform by incorporating more responsible AI features into the AI lifecycle for the benefit of end users.
Conclusion
While AI is a beacon of potential and transformative benefits, it is essential to remain cognizant of the accompanying risks. Platforms like DataRobot are pivotal in ensuring that the power of AI is harnessed responsibly, driving real-world value, while proactively addressing challenges.
1 The White House. n.d. “National AI Advisory Committee.” AI.Gov. https://ai.gov/naiac/.
2 “FINDINGS: The Potential Future Risks of AI.” October 2023. National Artificial Intelligence Advisory Committee (NAIAC). https://ai.gov/wp-content/uploads/2023/11/Findings_The-Potential-Future-Risks-of-AI.pdf.
3 “STATEMENT: On AI and Existential Risk.” October 2023. National Artificial Intelligence Advisory Committee (NAIAC). https://ai.gov/wp-content/uploads/2023/11/Statement_On-AI-and-Existential-Risk.pdf.
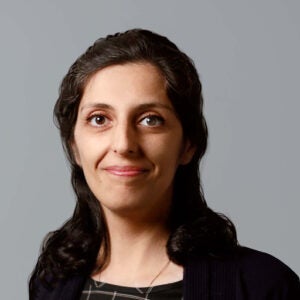
Global AI Ethicist, DataRobot
Haniyeh is a Global AI Ethicist at the DataRobot Trusted AI team and a member of the National AI Advisory Committee (NAIAC). Her research focuses on bias, privacy, robustness and stability, and ethics in AI and Machine Learning. She has a demonstrated history of implementing ML and AI in a variety of industries and initiated the incorporation of bias and fairness feature into DataRobot product. She is a thought leader in the area of AI bias and ethical AI. Haniyeh holds a PhD in Astronomy and Astrophysics from the Rheinische Friedrich-Wilhelms-Universität Bonn.
-
How to Choose the Right LLM for Your Use Case
April 18, 2024· 7 min read -
Belong @ DataRobot: Celebrating 2024 Women’s History Month with DataRobot AI Legends
March 28, 2024· 6 min read -
Choosing the Right Vector Embedding Model for Your Generative AI Use Case
March 7, 2024· 8 min read
Latest posts
Related Posts
You’ve just successfully subscribed